I was honored to particilate in a panel at an event on Adult Education in the Age of Artificial Intelligence that was run by The Great Courses as a fundraiser for the Academy of Hope, an adult public charter school in Washington, D.C.
I spoke first, following a few introductory talks, and was followed by Nicole Smith and Ellen Scully-Russ, and a keynote from Dexter Manley, Super Bowl winner with the Washington Redskins. After a short break, Kavitha Cardoza moderated a very interesting panel discussion. A recording of the talk and rest of the event is supposed to be available to Great Courses Plus subscribers.
I’ve included a fairly complete text from the script I wrote (with some modifications and additional comments).
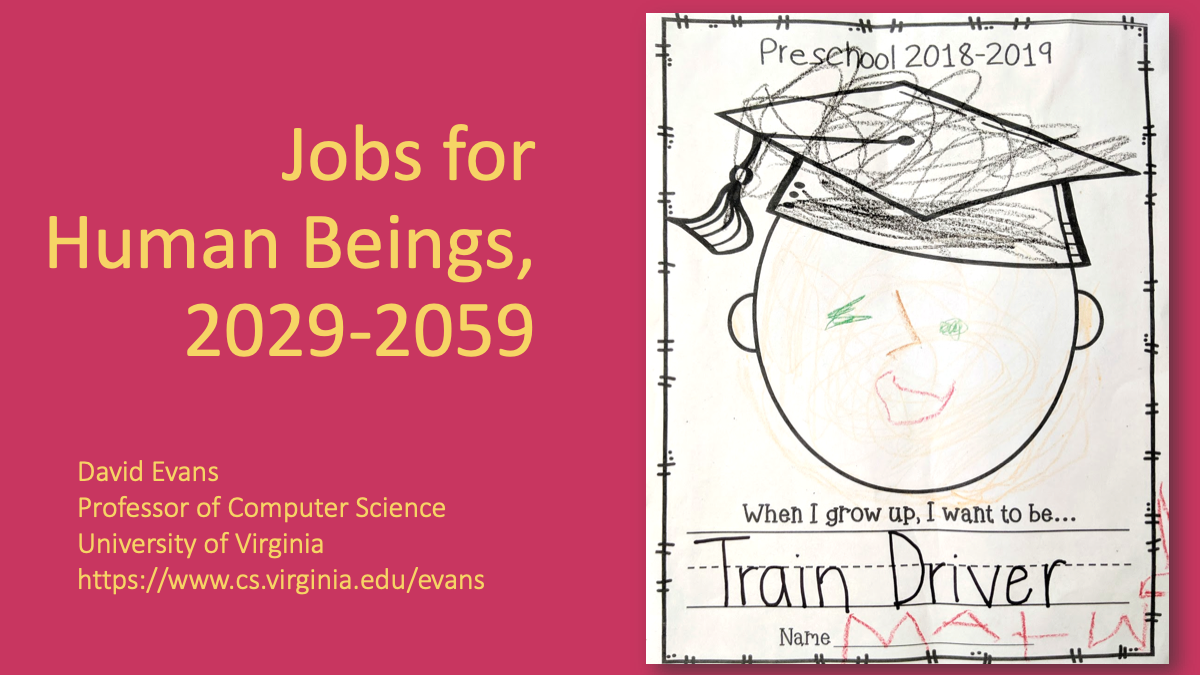
As a tenured professor at a well-endowed university, I have the good fortune to be about as sheltered as anyone can be from disruptions in employment. But, I do have two young children, so I have a strong personal interest in there being good jobs available for them in this time period.
My four-year old son drew the picture, and I hate to disappoint him that the train driver position he dreams of probably won’t work out, and I don’t think there is anything we can do to save it. But, I do hope there will be something fulfilling for him to do when he grows up.
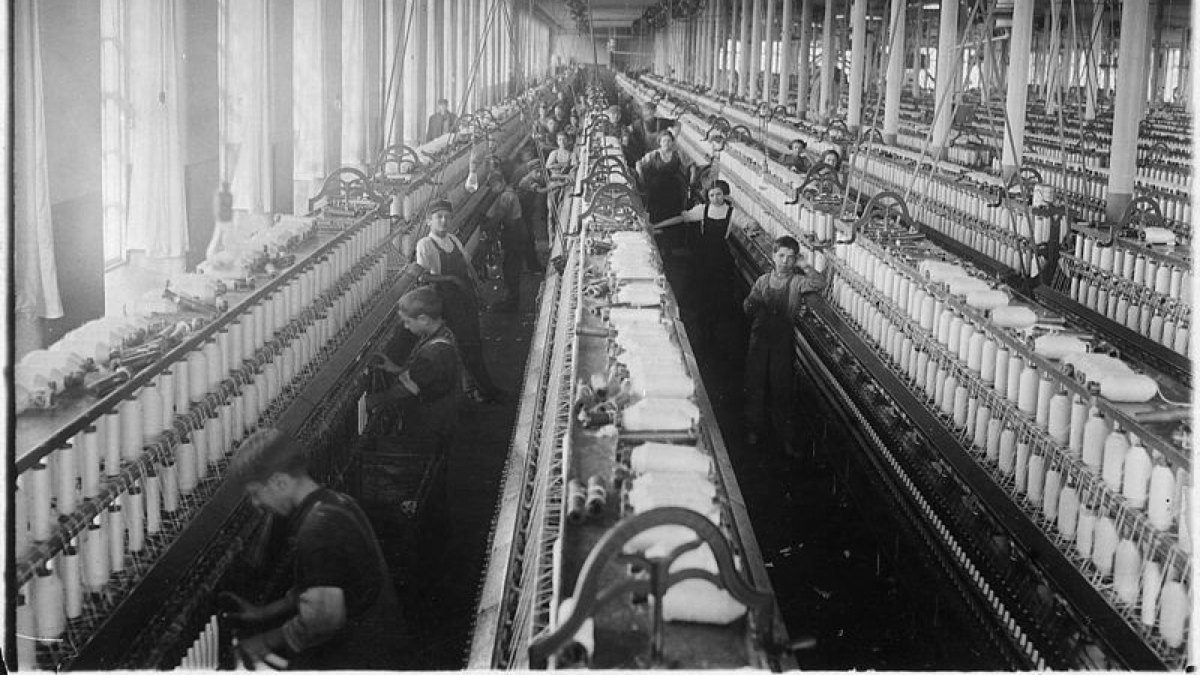
For the actual talk, I was able to use beautiful images from Getty Images which The Great Courses has a license to (and not allowed to use Create Commons images, since they do not count as non-commercial), so I’ve replaced the images from the talk with CC-licensed images. Unfortunately, I wasn’t able to find a cc image of a match making factory, like the one in this Getty image that I used in the talk. The replacement image is a spinning room from Fall River, MA, 1912.
I want to start by talking about history. Machines taking jobs away from humans is not a new thing - goes back hundreds of years.
The picture shows a match-making factory in the 1870s. No one has a job as this kind of match-maker today – and lots of the jobs the other kind of match-maker have also been taken over by machines.
ATM Machines didn’t eliminate jobs for bank tellers – actually made more, since they make banking cheaper and more accessible, and meant banks could open more branches which still needed to hire tellers for the more interesting and complicated banking activities.
We’ve seen many transformations like this, and many tasks that could once only be done by humans are now routinely automated. Although past technological advances have caused massive disruption and pain for many individuals, as a species on the whole, these advances have not diminished overall human employment, and there shouldn’t be any doubt that on the whole, technological and scientific program have made all of our lives tremendously better.
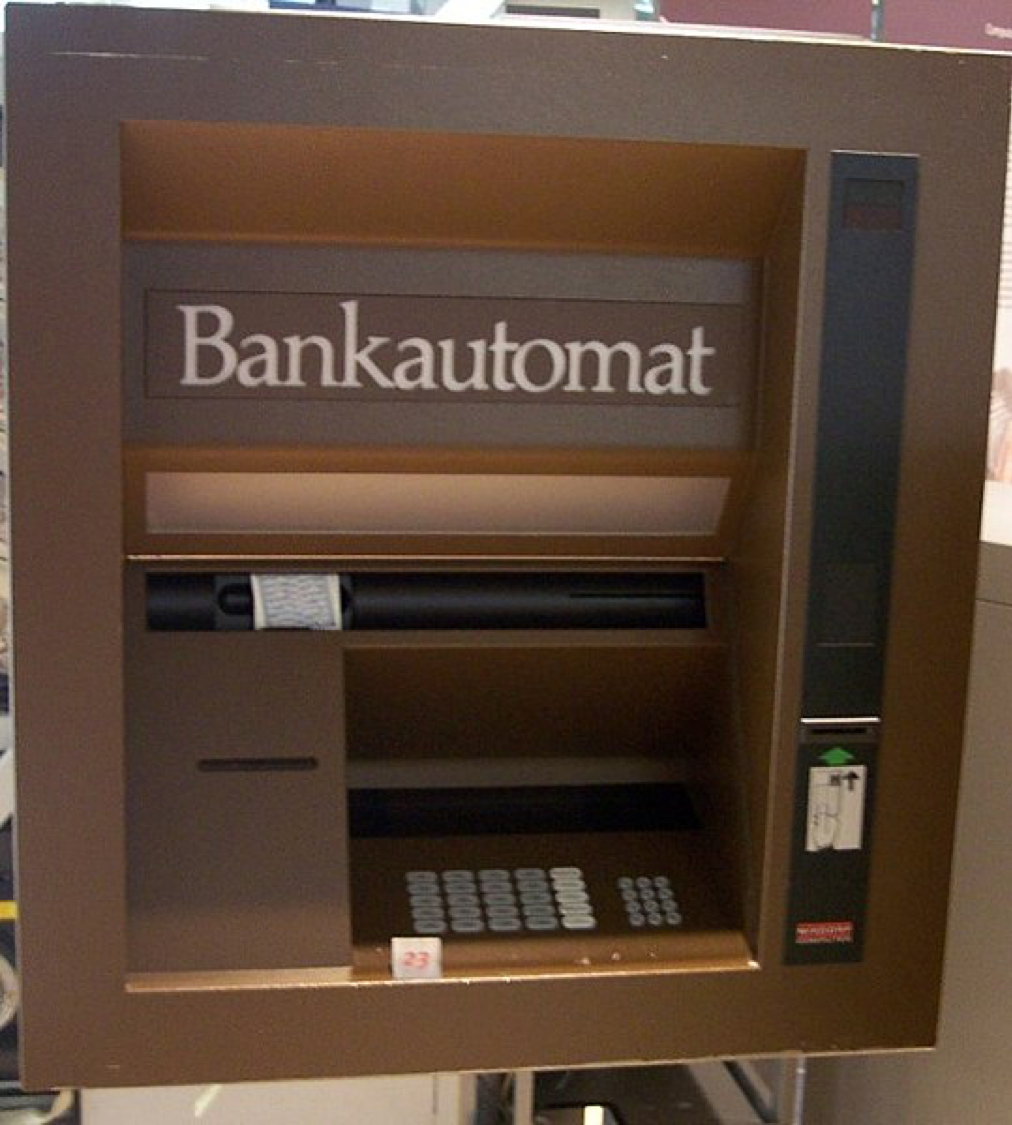
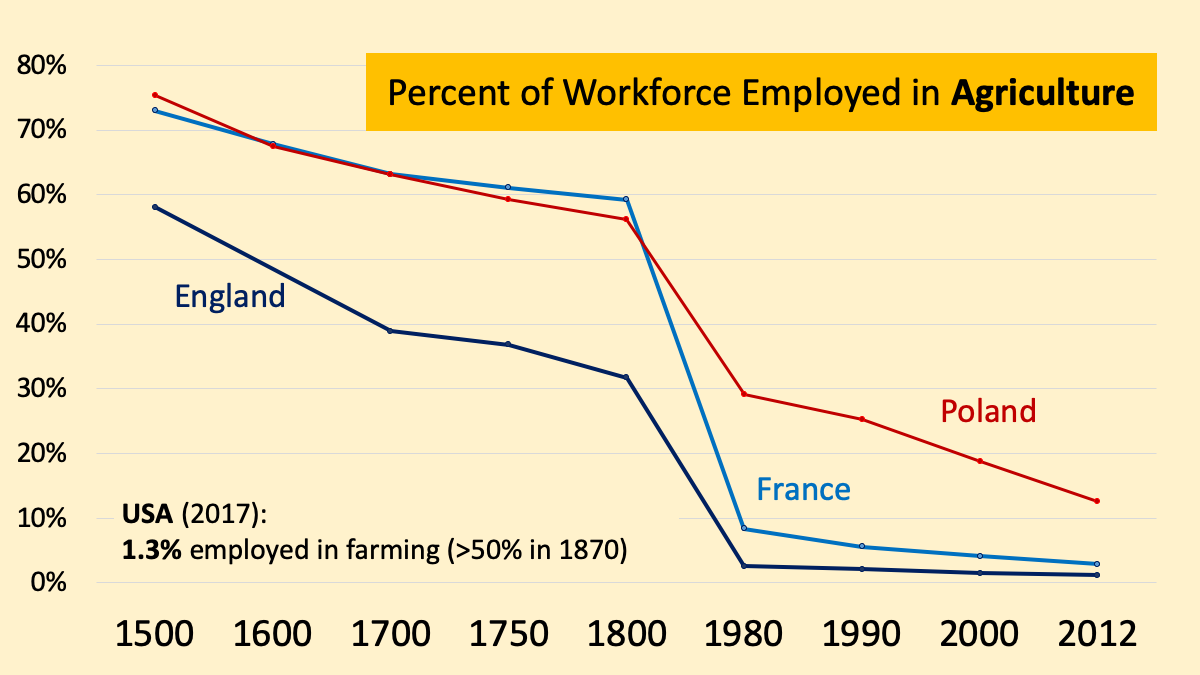
One way to measure that progress is what fraction of our workforce is needed to feed us.
As recently as 150 years ago, nearly everyone worked in agriculture, and we still couldn’t produce enough food to feed everyone. Now, only about 1 out of 75 people work in farming, and we are so ridiculously productive in producing food with we can burn about 1/3 of it as fuel.
So, if we were driven as a species to avoid work once our subsistence needs are met, we shouldn’t be working 8 hours or more a day.
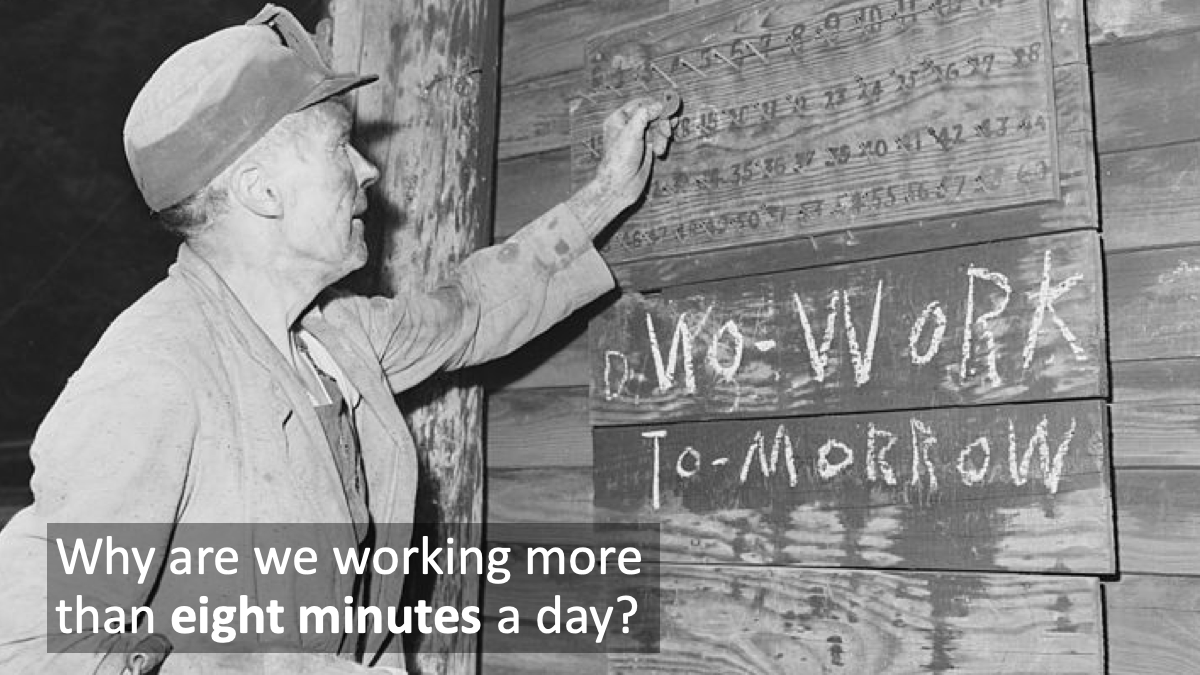
Bertrand Russell, In Praise of Idleness (1932)
Somehow we’ve managed improve productivity by a factor of several hundred, without reducing the demand for human work. All the previous scientific and technological progress that has automated work has just led to finding new productive things for humans to do.
Will Artificial Intelligence Change Everything?
The question for today is if we are reaching the end of that – are the kinds of automation that can be achieved (or soon will be) with artificial intelligence that they won’t just improve productivity by automating some human tasks, but that they will eliminate the opportunity for humans to contribute productively at all.
First, its important to distinguish AI from the traditional automation that has driven productivity gains for the past hundred years.
We’ve only experienced the disruption computing causes over the past half century, but a few people had the vision of what was possible much longer ago.
The first person to see the potential for universal computers, was Gottfried Leibniz back in the 17th century.
Gottfried Wilhelm Leibniz (1679)
Leibniz is talking about increasing the power of our minds the way a telescope increases the power of our eyes. This is what traditional computing has done.
More recently, Steve Jobs talked about computers as bicycles for the mind.
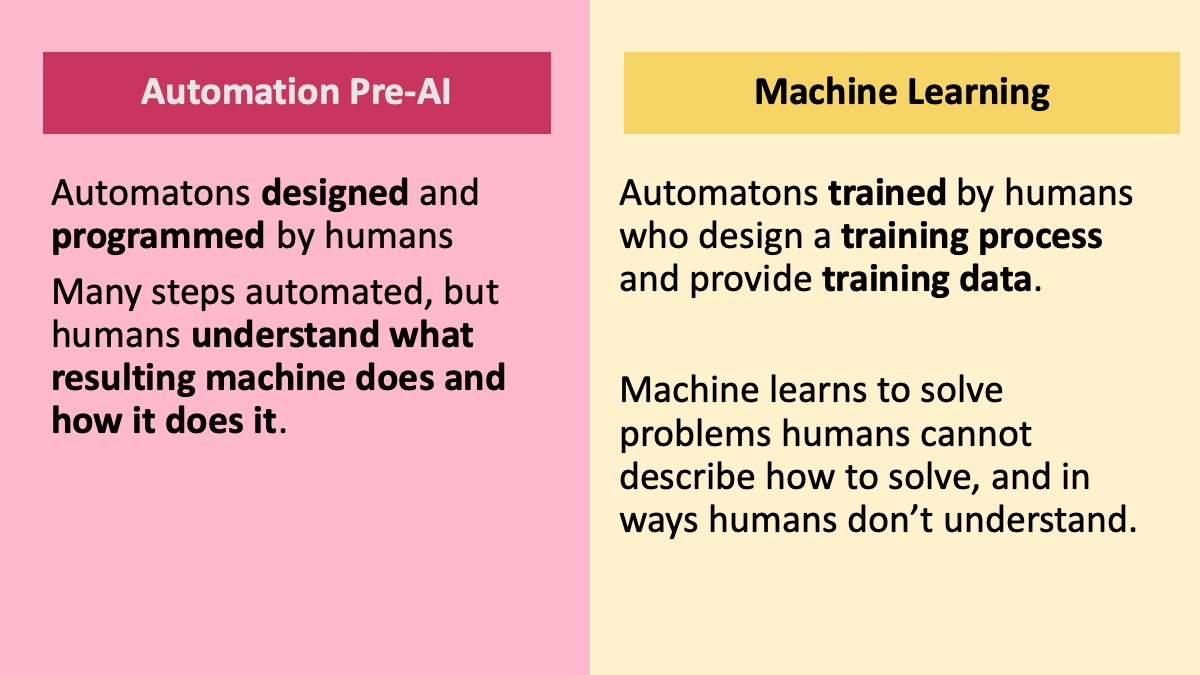
All of the previous capabilities can only solve problems humans already understand well enough to solve.
AI allows us to solve problems no humans understand.
I’m going to focus now on Machine Learning, which is just one sub-field of AI, but it’s the one that has the most hype over the past decade, and one that is being rapidly deployed in ways that automate human tasks.
The main distinction between traditional computing and machine learning is that traditional automatons are programmed, while machine learning automatons are trained. Humans set up a training process, but the machine learns how to solve the problem on its own.
This is something most humans are quite good at, but if I asked you how you do it, you couldn’t explain it. And, since humans can’t explain how to do it, we don’t know how to write a program to do it, but we can train a model that does it well.
Training complex models requires a lot of training data – a few million images. Fortunately, you can find billions of images on the Internet (at least if you don’t care about copyright; for the talk, I was allowed to use these images, but they blurred them out of the recording).
Labeling 10M images might seem like a lot of work, but you can find low-paid humans to do it.
This just means designing a function with lots of unknown parameters that takes all the pixels of an images as its inputs, and outputs a prediction of gender and confidence.
There are some tricks for how to update the weights in the right direction, but if all goes well, we’ll find a model that has high accuracy on our tests before we run out of money.
Then we deploy the model and everything is great...
...except we see results like these.
We have people that look like men, but are identified as women. What they have in common is they are carrying umbrellas.
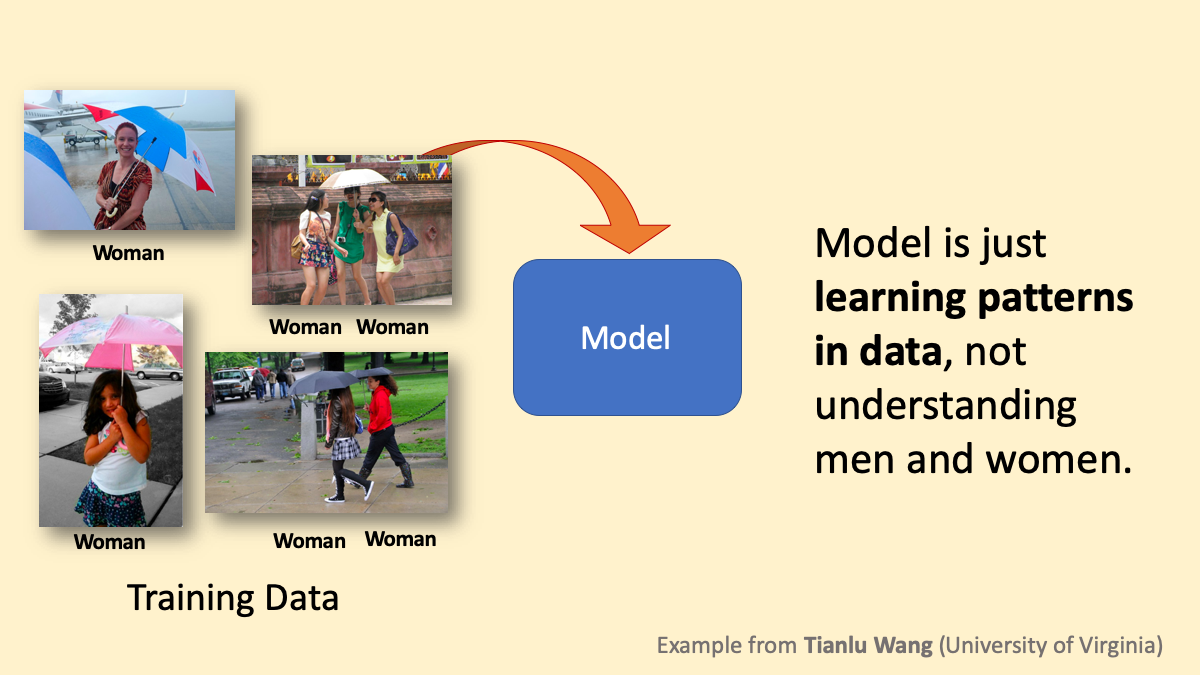
The umbrella-revealing-gender example is from Balanced Datasets Are Not Enough: Estimating and Mitigating Gender Bias in Deep Image Representations by Tianlu Wang, Jieyu Zhao, Mark Yatskar, Kai-Wei Chang, Vicente Ordonez. (In International Conference on Computer Vision (ICCV), 2019.)
Since most of the people in the labeled data who were holding umbrellas were women, the model learned a strong pattern that if you are holding an umbrella you must be a woman.
Being mis-gendered because of holding an umbrella may not be such a serious problem, but the same kinds of learning methods are used in many more sensitive tasks, like predicting who is a terrorist and who gets a job interview.
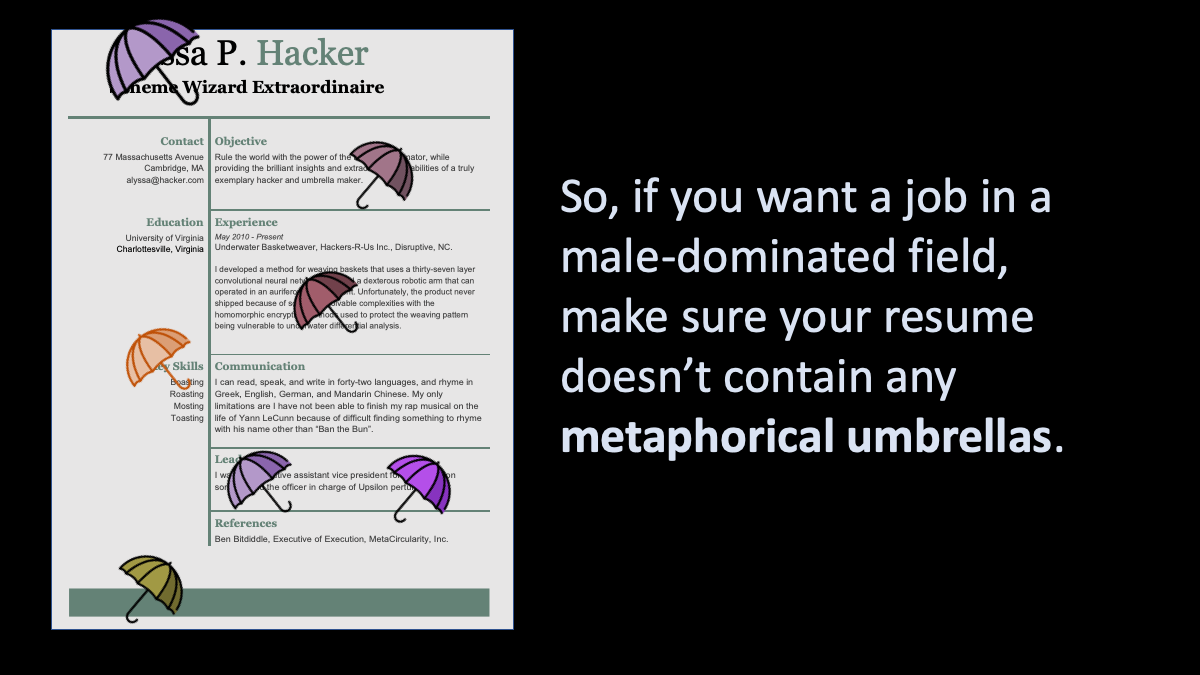
Amazon Created a Hiring Tool Using A.I. It Immediately Started Discriminating Against Women. (Slate, Oct 2018)
If you are traveling in Hong Kong, being face-recognized with an umbrella nearby may lead to other problems.