USENIX Security Symposium 2019
Bargav Jayaraman presented our paper on Evaluating Differentially Private Machine Learning in Practice at the 28th USENIX Security Symposium in Santa Clara, California.
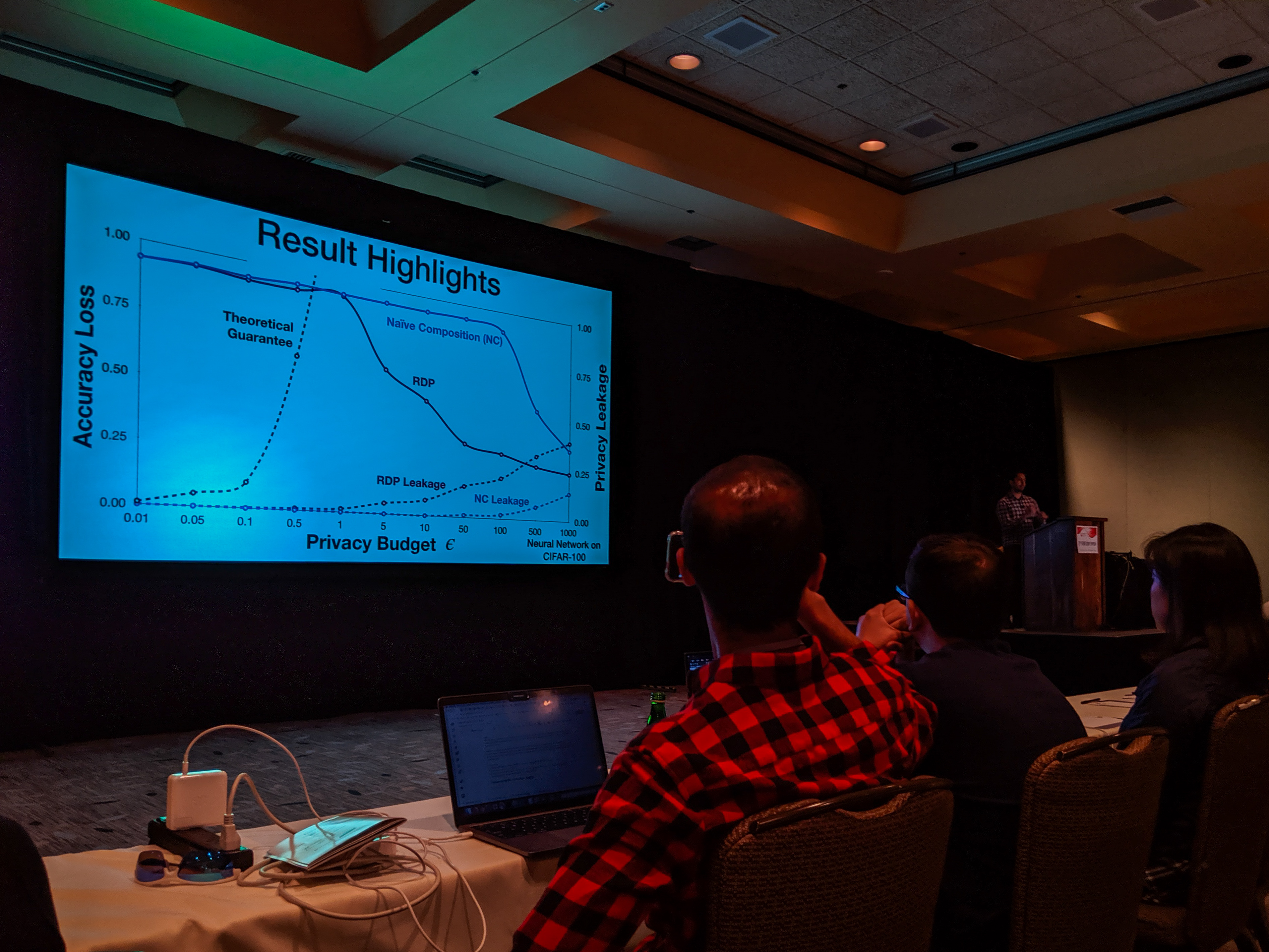
Summary by Lea Kissner:
Hey it's the results! pic.twitter.com/ru1FbkESho
— Lea Kissner (@LeaKissner) August 17, 2019
Also, great to see several UVA folks at the conference including:
- Sam Havron (BSCS 2017, now a PhD student at Cornell) presented a paper on the work he and his colleagues have done on computer security for victims of intimate partner violence.
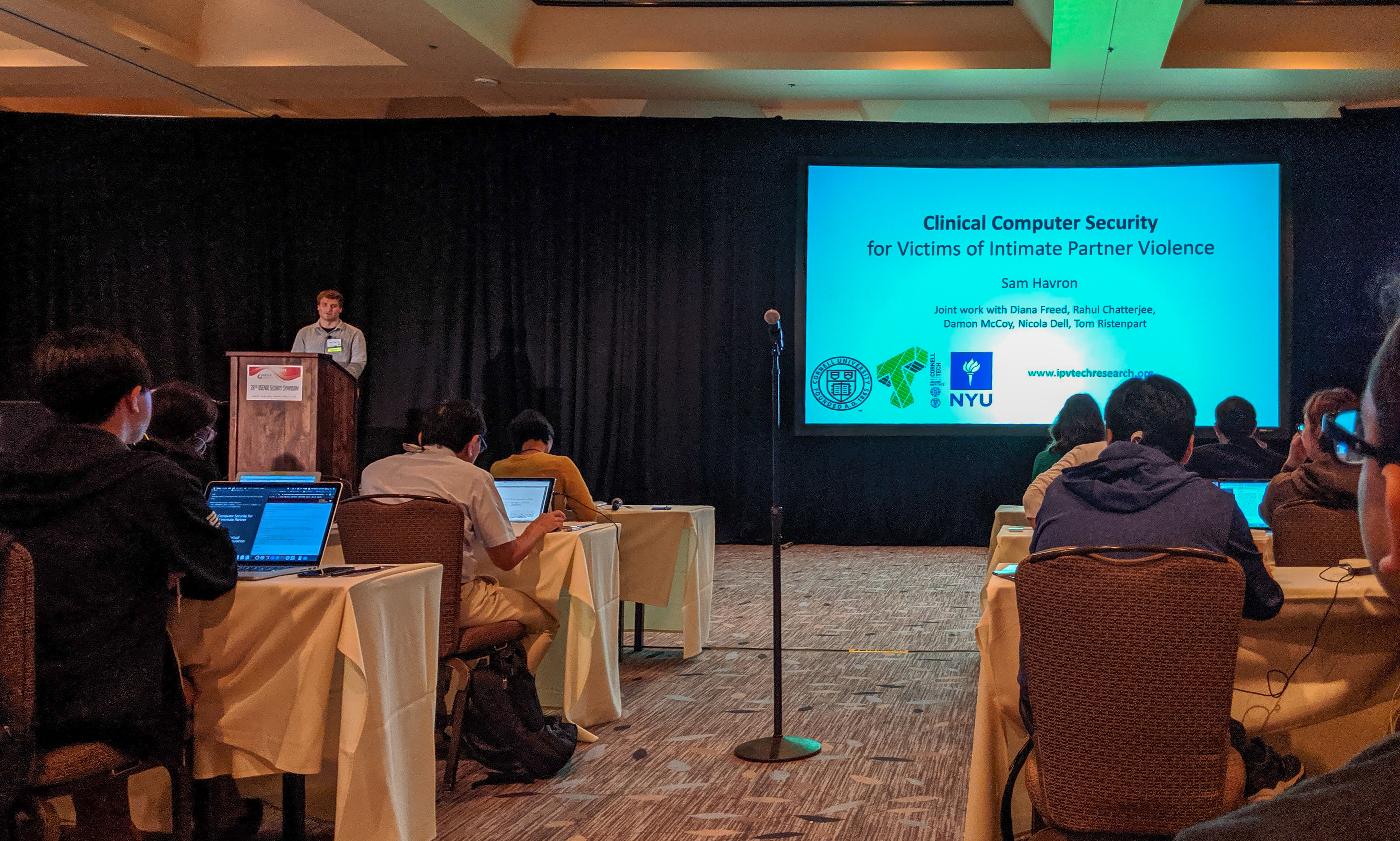
-
Serge Egelman (BSCS 2004) was an author on the paper 50 Ways to Leak Your Data: An Exploration of Apps’ Circumvention of the Android Permissions System (which was recognized by a Distinguished Paper Award). His paper in SOUPS on Privacy and Security Threat Models and Mitigation Strategies of Older Adults was highlighted in Alex Stamos’ excellent talk.
NeurIPS 2018: Distributed Learning without Distress
Bargav Jayaraman presented our work on privacy-preserving machine learning at the 32nd Conference on Neural Information Processing Systems (NeurIPS 2018) in Montreal.
Distributed learning (sometimes known as federated learning) allows a group of independent data owners to collaboratively learn a model over their data sets without exposing their private data. Our approach combines differential privacy with secure multi-party computation to both protect the data during training and produce a model that provides privacy against inference attacks.