Anshuman Suri and Pratyush Maini wrote a blog about the EMNLP 2024 best paper award winner: Reassessing EMNLP 2024’s Best Paper: Does Divergence-Based Calibration for Membership Inference Attacks Hold Up?.
As we explored in Do Membership Inference Attacks Work on Large Language Models?, to test a membership inference attack it is essentail to have a candidate set where the members and non-members are from the same distribution. If the distributions are different, the ability of an attack to distinguish members and non-members is indicative of distribution inference, not necessarily membership inference.
Read More…
UVA News has an article on our LLM membership inference work:
Common Way To Test for Leaks in Large Language Models May Be Flawed: UVA Researchers Collaborated To Study the Effectiveness of Membership Inference Attacks, Eric Williamson, 13 November 2024.
Matt Stoller published my essay on Google’s decision to abandon its Privacy Sandbox Initiative in his Big newsletter:
Google's Trail of Crumbs by Matt Stoller
Google is too big to get rid of cookies. Even when it wants to protect users, it can't.
Read on Substack
For more technical background on this, see Minjun’s paper: Evaluating Google’s Protected Audience Protocol in PETS 2024.
Congratulations to Anshuman Suri for successfully defending his PhD thesis!
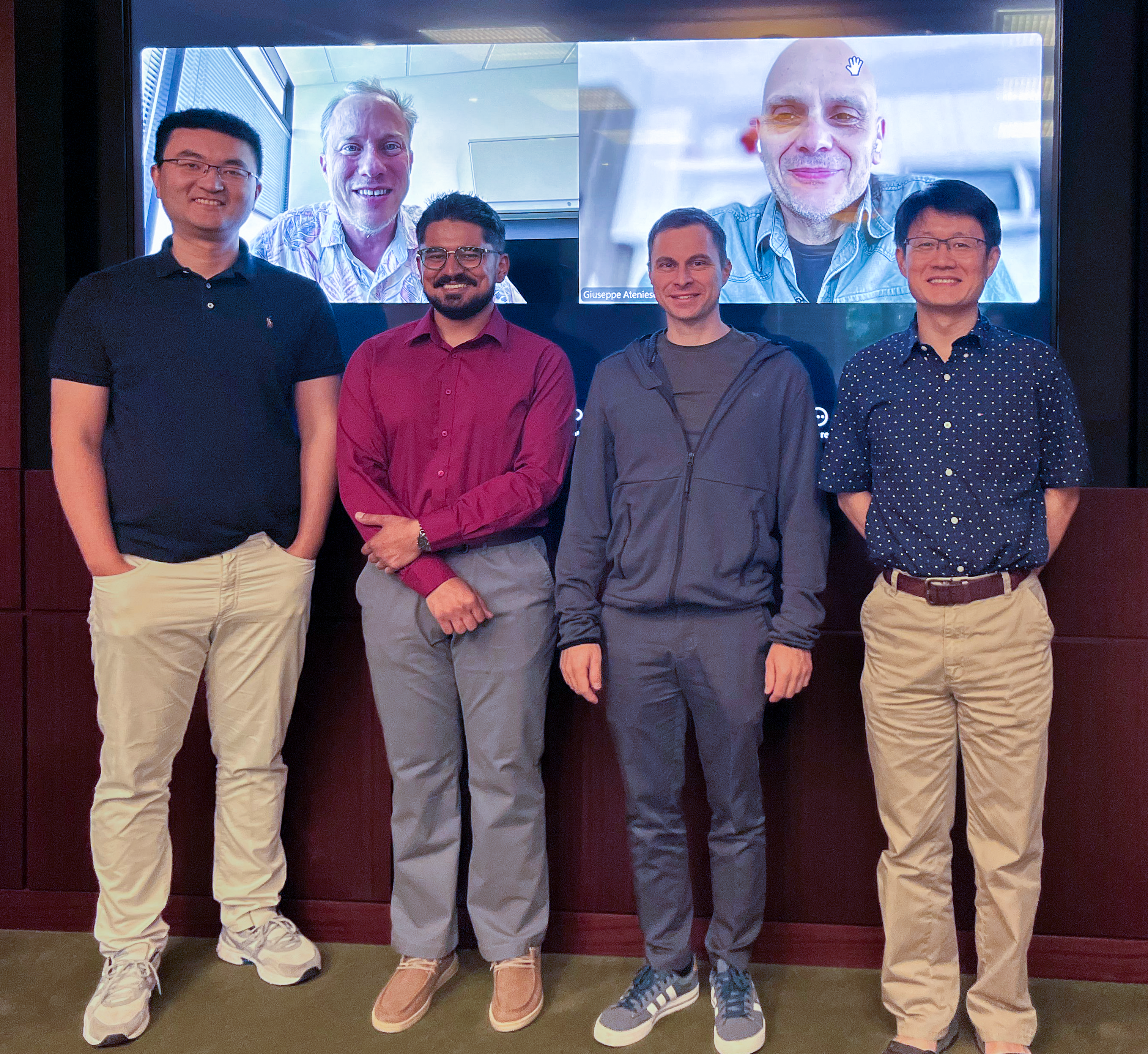
Tianhao Wang, Dr. Anshuman Suri, Nando Fioretto, Cong Shen
On Screen: David Evans, Giuseppe Ateniese
Inference Privacy in Machine Learning
Using machine learning models comes at the risk of leaking information about data used in their training and deployment. This leakage can expose sensitive information about properties of the underlying data distribution, data from participating users, or even individual records in the training data. In this dissertation, we develop and evaluate novel methods to quantify and audit such information disclosure at three granularities: distribution, user, and record.
Read More…
Congratulations to our two PhD graduates!
Suya will be joining the University of Tennessee at Knoxville as an Assistant Professor.
Josie will be building a medical analytics research group at Dexcom.
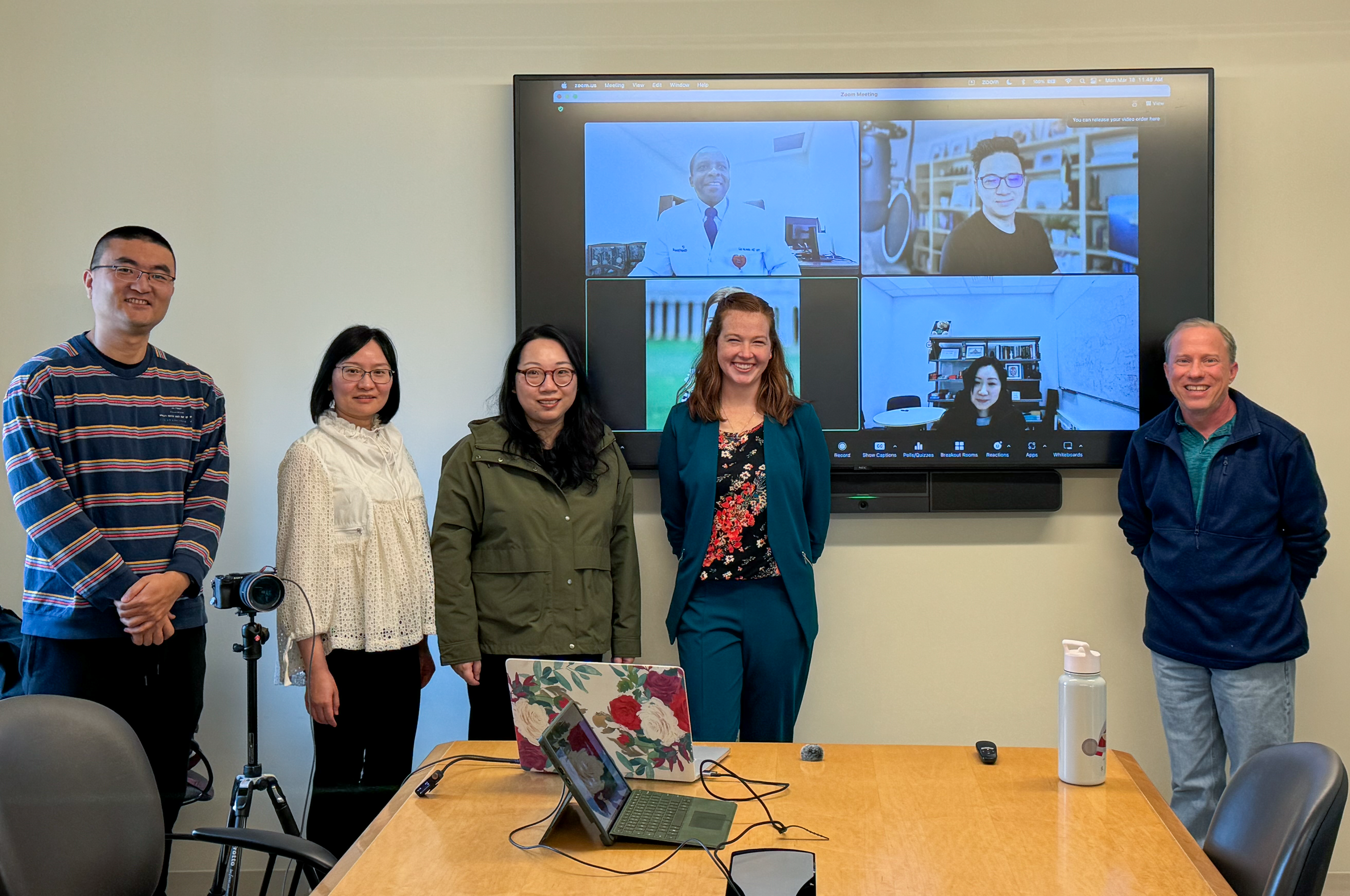
Tianhao Wang (Committee Chair), Miaomiao Zhang, Lu Feng (Co-Advisor), Dr. Josie Lamp, David Evans
On screen: Sula Mazimba, Rich Nguyen, Tingting Zhu
Congratulations to Josephine Lamp for successfully defending her PhD thesis!
Trustworthy Clinical Decision Support Systems for Medical Trajectories
The explosion of medical sensors and wearable devices has resulted in the collection of large amounts of medical trajectories. Medical trajectories are time series that provide a nuanced look into patient conditions and their changes over time, allowing for a more fine-grained understanding of patient health. It is difficult for clinicians and patients to effectively make use of such high dimensional data, especially given the fact that there may be years or even decades worth of data per patient. Clinical Decision Support Systems (CDSS) provide summarized, filtered, and timely information to patients or clinicians to help inform medical decision-making processes. Although CDSS have shown promise for data sources such as tabular and imaging data, e.g., in electronic health records, the opportunities of CDSS using medical trajectories have not yet been realized due to challenges surrounding data use, model trust and interpretability, and privacy and legal concerns.
Read More…
Here are the slides for my talk at the Practical and Theoretical Privacy of Machine Learning Training Pipelines
Workshop at the Microsoft Research Summit (21 October 2021):
Surprising (and Unsurprising) Inference Risks in Machine Learning [PDF]
The work by Bargav Jayaraman (with Katherine Knipmeyer, Lingxiao Wang,
and Quanquan Gu) that I talked about on improving membership inference
attacks is described in more details here:
-
Bargav Jayaraman, Lingxiao Wang, Katherine Knipmeyer, Quanquan Gu, David Evans. Revisiting Membership Inference Under Realistic Assumptions (PETS 2021).
[Blog] [Code: https://github.com/bargavj/EvaluatingDPML]
Read More…
UVA News has an article by Audra Book on our research on security and
privacy of machine learning (with some very nice quotes from several
students in the group, and me saying something positive about the
NSA!): Computer science professor David Evans and his team conduct
experiments to understand security and privacy risks associated with
machine
learning,
8 September 2021.
David Evans, professor of computer science in the University of Virginia School of Engineering and Applied Science, is leading research to understand how machine learning models can be compromised.
Read More…
I gave an invited talk at the Distributed and Private Machine Learning (DPML) workshop at ICLR 2021 on Inference Risks for Machine Learning.
The talk mostly covers work by Bargav Jayaraman on evaluating privacy in
machine learning and connecting attribute inference and imputation, and recent work by Anshuman Suri on property inference.
Here are the slides for my talk at the
11th ACM Conference on Data and Application Security and Privacy:
When Models Learn Too Much [PDF]
The talk includes Bargav Jayaraman’s work (with Katherine Knipmeyer, Lingxiao Wang, and Quanquan Gu) on evaluating privacy in machine learning, as well as more recent work by Anshuman Suri on property inference attacks, and Bargav on attribute inference and imputation: