Reassessing EMNLP 2024’s Best Paper: Does Divergence-Based Calibration for Membership Inference Attacks Hold Up?
Anshuman Suri and Pratyush Maini wrote a blog about the EMNLP 2024 best paper award winner: Reassessing EMNLP 2024’s Best Paper: Does Divergence-Based Calibration for Membership Inference Attacks Hold Up?. As we explored in Do Membership Inference Attacks Work on Large Language Models?, to test a membership inference attack it is essentail to have a candidate set where the members and non-members are from the same distribution. If the distributions are different, the ability of an attack to distinguish members and non-members is indicative of distribution inference, not necessarily membership inference.Common Way To Test for Leaks in Large Language Models May Be Flawed
UVA News has an article on our LLM membership inference work: Common Way To Test for Leaks in Large Language Models May Be Flawed: UVA Researchers Collaborated To Study the Effectiveness of Membership Inference Attacks, Eric Williamson, 13 November 2024.
The Mismeasure of Man and Models
Evaluating Allocational Harms in Large Language Models Blog post written by Hannah Chen Our work considers allocational harms that arise when model predictions are used to distribute scarce resources or opportunities. Current Bias Metrics Do Not Reliably Reflect Allocation Disparities Several methods have been proposed to audit large language models (LLMs) for bias when used in critical decision-making, such as resume screening for hiring. Yet, these methods focus on predictions, without considering how the predictions are used to make decisions.Google's Trail of Crumbs
Matt Stoller published my essay on Google’s decision to abandon its Privacy Sandbox Initiative in his Big newsletter:
For more technical background on this, see Minjun’s paper: Evaluating Google’s Protected Audience Protocol in PETS 2024.
Technology: US authorities survey AI ecosystem through antitrust lens
I’m quoted in this article for the International Bar Association: Technology: US authorities survey AI ecosystem through antitrust lens William Roberts, IBA US Correspondent Friday 2 August 2024 Antitrust authorities in the US are targeting the new frontier of artificial intelligence (AI) for potential enforcement action. … Jonathan Kanter, Assistant Attorney General for the Antitrust Division of the DoJ, warns that the government sees ‘structures and trends in AI that should give us pause’.John Guttag Birthday Celebration
Maggie Makar organized a celebration for the 75th birthday of my PhD advisor, John Guttag.
I wasn’t able to attend in person, unfortunately, but the occasion provided an opportunity to create a poster that looks back on what I’ve done since I started working with John over 30 years ago.
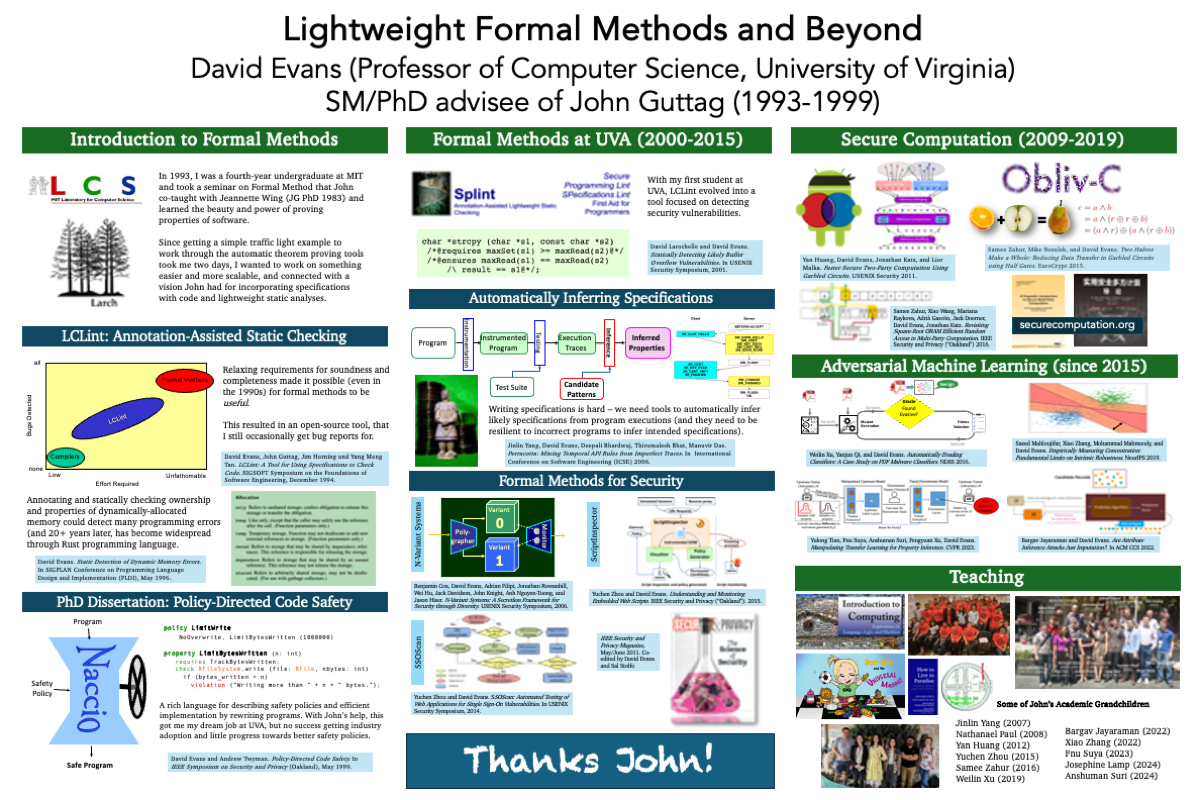
SaTML Talk: SoK: Pitfalls in Evaluating Black-Box Attacks
Anshuman Suri’s talk at IEEE Conference on Secure and Trustworthy Machine Learning (SaTML) is now available:
See the earlier blog post for more on the work, and the paper at https://arxiv.org/abs/2310.17534.