Congratulations, Dr. Suri!
Congratulations to Anshuman Suri for successfully defending his PhD thesis!
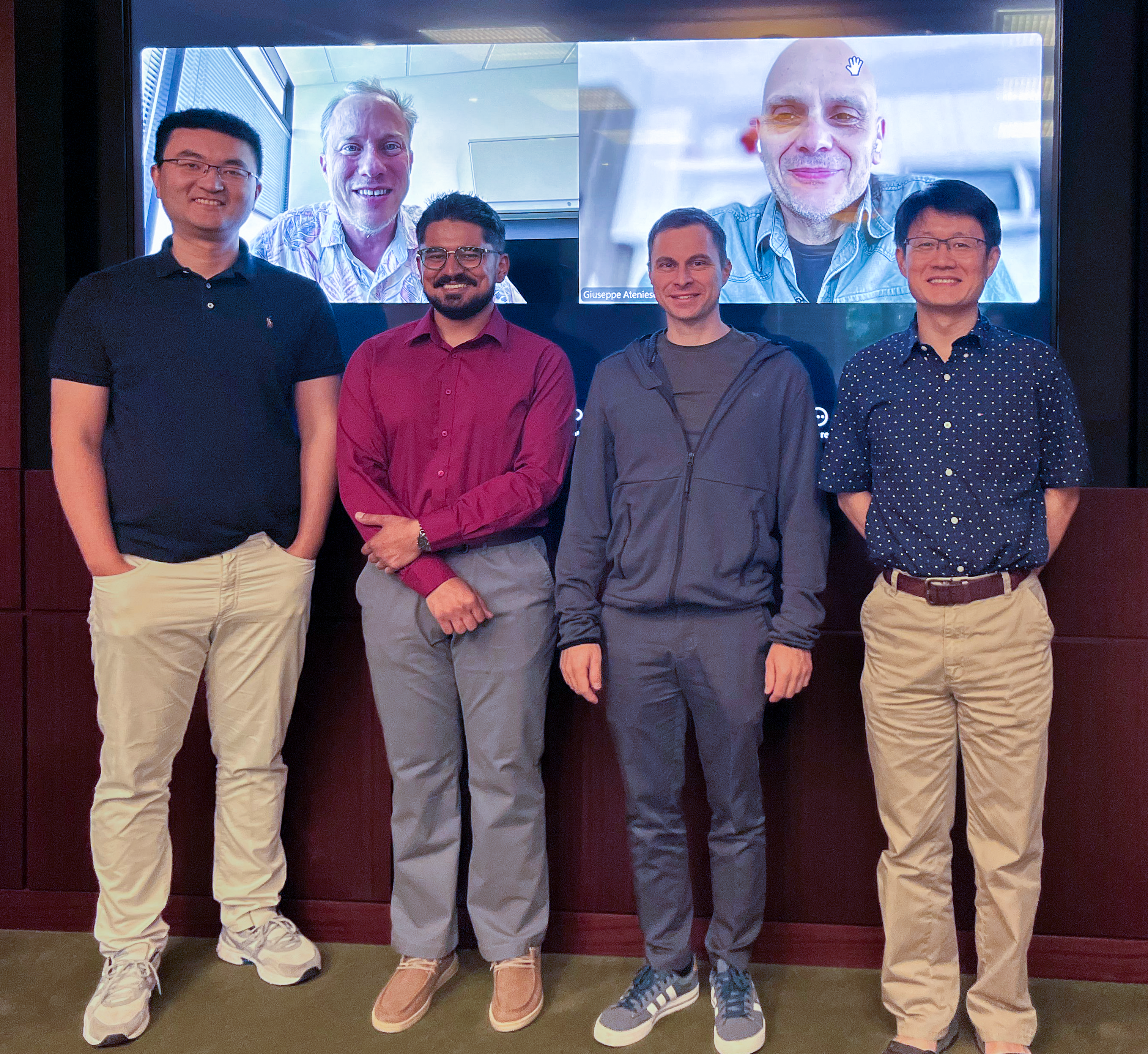
Tianhao Wang, Dr. Anshuman Suri, Nando Fioretto, Cong Shen
On Screen: David Evans, Giuseppe Ateniese
Using machine learning models comes at the risk of leaking information about data used in their training and deployment. This leakage can expose sensitive information about properties of the underlying data distribution, data from participating users, or even individual records in the training data. In this dissertation, we develop and evaluate novel methods to quantify and audit such information disclosure at three granularities: distribution, user, and record.
Congratulations, Dr. Lamp!
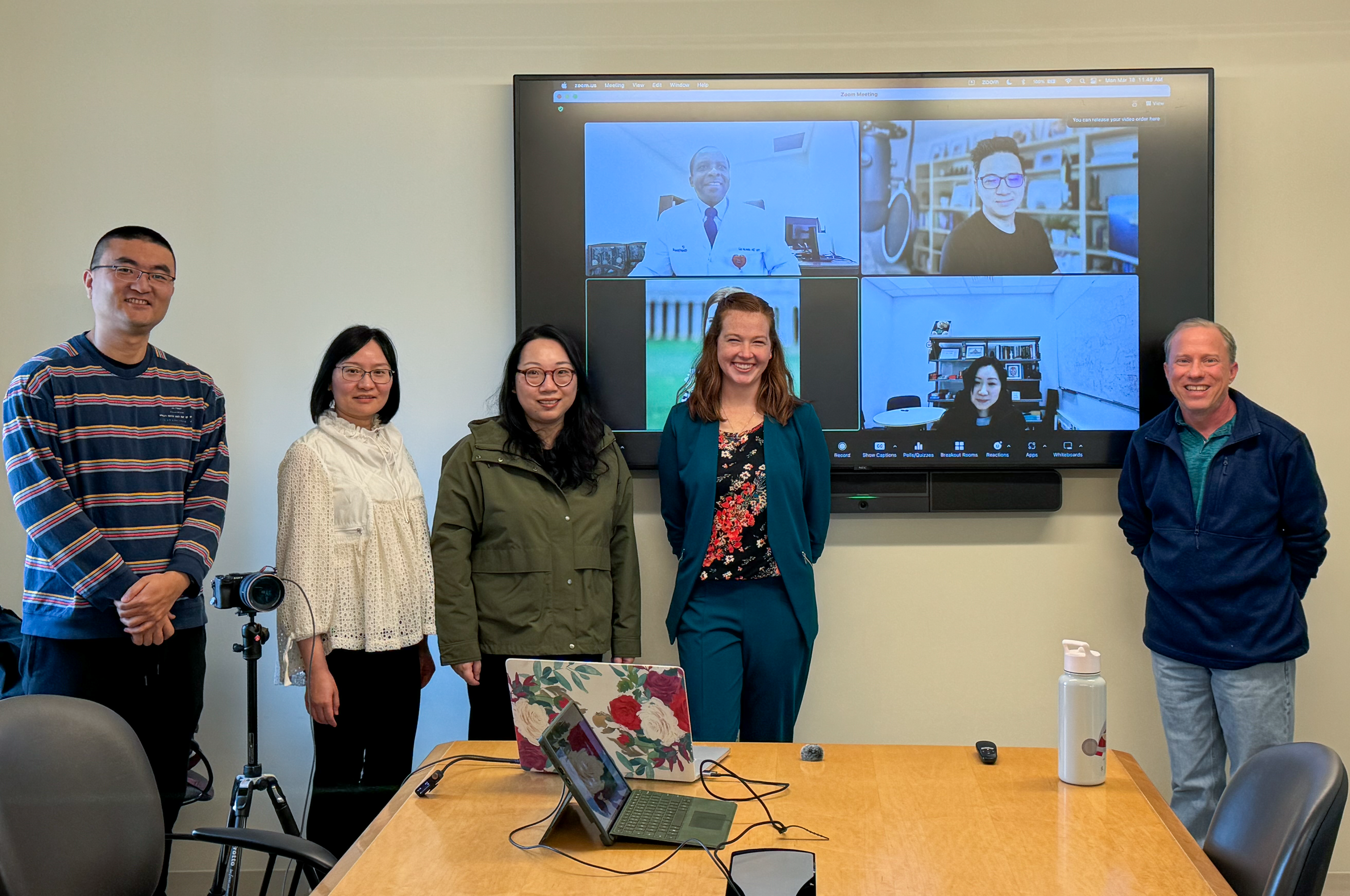
Tianhao Wang (Committee Chair), Miaomiao Zhang, Lu Feng (Co-Advisor), Dr. Josie Lamp, David Evans
On screen: Sula Mazimba, Rich Nguyen, Tingting Zhu
Congratulations to Josephine Lamp for successfully defending her PhD thesis!
The explosion of medical sensors and wearable devices has resulted in the collection of large amounts of medical trajectories. Medical trajectories are time series that provide a nuanced look into patient conditions and their changes over time, allowing for a more fine-grained understanding of patient health. It is difficult for clinicians and patients to effectively make use of such high dimensional data, especially given the fact that there may be years or even decades worth of data per patient. Clinical Decision Support Systems (CDSS) provide summarized, filtered, and timely information to patients or clinicians to help inform medical decision-making processes. Although CDSS have shown promise for data sources such as tabular and imaging data, e.g., in electronic health records, the opportunities of CDSS using medical trajectories have not yet been realized due to challenges surrounding data use, model trust and interpretability, and privacy and legal concerns.
Congratulations, Dr. Suya!
Congratulations to Fnu Suya for successfully defending his PhD thesis!
Suya will join the Unversity of Maryland as a MC2 Postdoctoral Fellow at the Maryland Cybersecurity Center this fall.
Current machine learning models require large amounts of labeled training data, which are often collected from untrusted sources. Models trained on these potentially manipulated data points are prone to data poisoning attacks. My research aims to gain a deeper understanding on the limits of two types of data poisoning attacks: indiscriminate poisoning attacks, where the attacker aims to increase the test error on the entire dataset; and subpopulation poisoning attacks, where the attacker aims to increase the test error on a defined subset of the distribution. We first present an empirical poisoning attack that encodes the attack objectives into target models and then generates poisoning points that induce the target models (and hence the encoded objectives) with provable convergence. This attack achieves state-of-the-art performance for a diverse set of attack objectives and quantifies a lower bound to the performance of best possible poisoning attacks. In the broader sense, because the attack guarantees convergence to the target model which encodes the desired attack objective, our attack can also be applied to objectives related to other trustworthy aspects (e.g., privacy, fairness) of machine learning.
Congratulations, Dr. Jayaraman!
Congratulations to Bargav Jayaraman for successfully defending his PhD thesis!
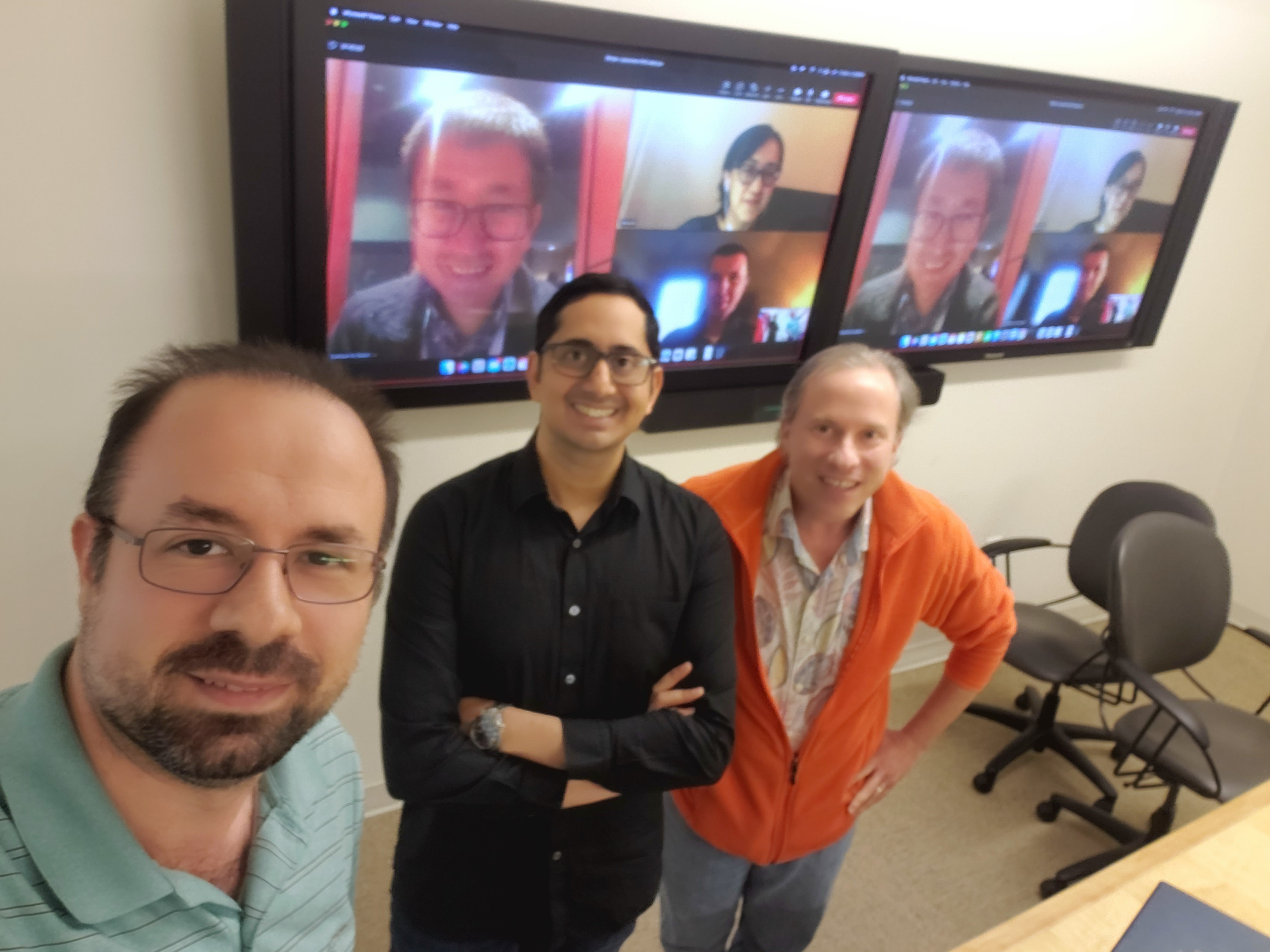
Bargav will join the Meta AI Lab in Menlo Park, CA as a post-doctoral researcher.
Machine learning models have been shown to leak sensitive information about their training data. An adversary having access to the model can infer different types of sensitive information, such as learning if a particular individual’s data is in the training set, extracting sensitive patterns like passwords in the training set, or predicting missing sensitive attribute values for partially known training records. This dissertation quantifies this privacy leakage. We explore inference attacks against machine learning models including membership inference, pattern extraction, and attribute inference. While our attacks give an empirical lower bound on the privacy leakage, we also provide a theoretical upper bound on the privacy leakage metrics. Our experiments across various real-world data sets show that the membership inference attacks can infer a subset of candidate training records with high attack precision, even in challenging cases where the adversary’s candidate set is mostly non-training records. In our pattern extraction experiments, we show that an adversary is able to recover email ids, passwords and login credentials from large transformer-based language models. Our attribute inference adversary is able to use underlying training distribution information inferred from the model to confidently identify candidate records with sensitive attribute values. We further evaluate the privacy risk implication to individuals contributing their data for model training. Our findings suggest that different subsets of individuals are vulnerable to different membership inference attacks, and that some individuals are repeatedly identified across multiple runs of an attack. For attribute inference, we find that a subset of candidate records with a sensitive attribute value are correctly predicted by our white-box attribute inference attacks but would be misclassified by an imputation attack that does not have access to the target model. We explore different defense strategies to mitigate the inference risks, including approaches that avoid model overfitting such as early stopping and differential privacy, and approaches that remove sensitive data from the training. We find that differential privacy mechanisms can thwart membership inference and pattern extraction attacks, but even differential privacy fails to mitigate the attribute inference risks since the attribute inference attack relies on the distribution information leaked by the model whereas differential privacy provides no protection against leakage of distribution statistics.
Congratulations, Dr. Zhang!
Congratulations to Xiao Zhang for successfully defending his PhD thesis!
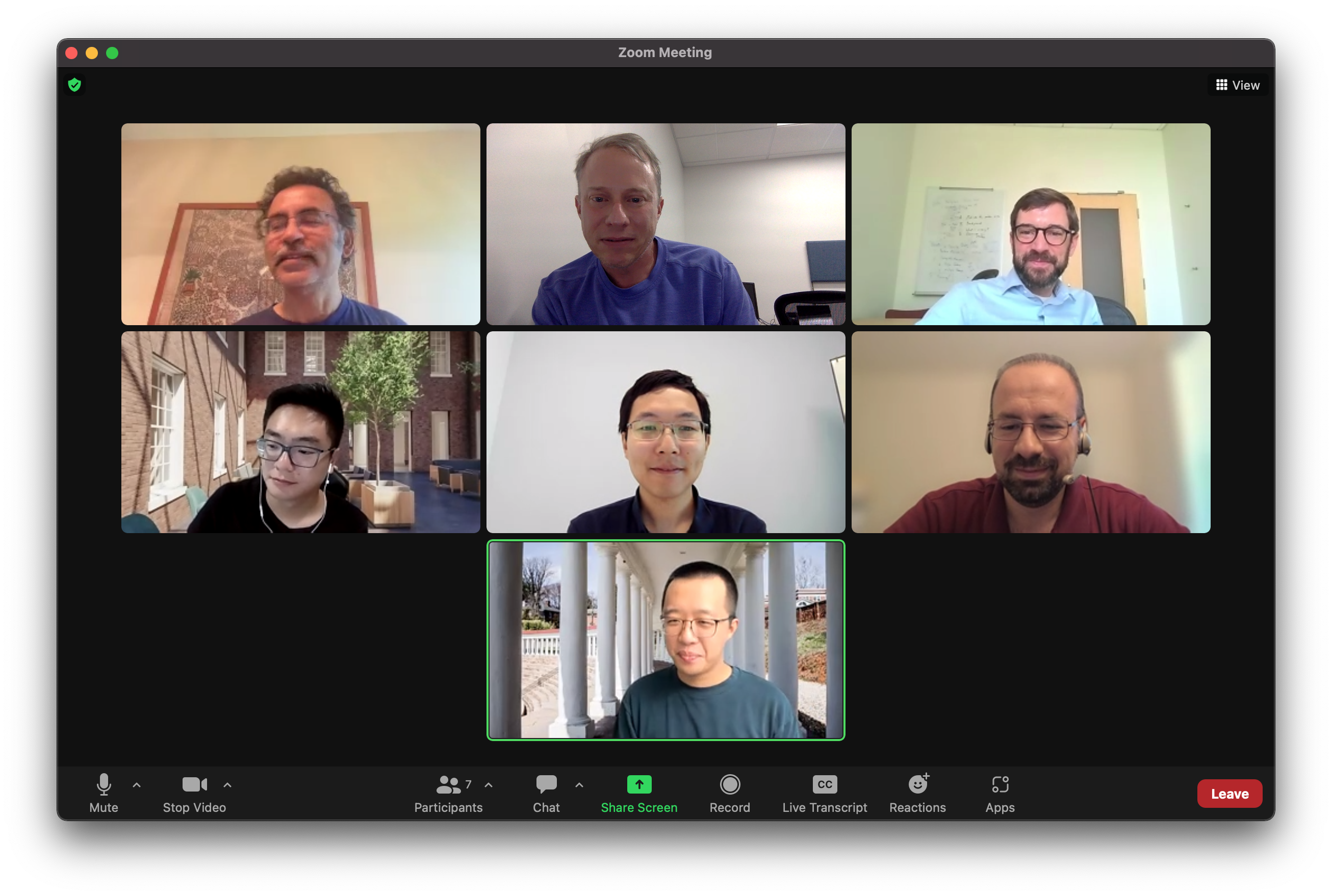
Xiao will join the CISPA Helmholtz Center for Information Security in Saarbrücken, Germany this fall as a tenure-track faculty member.
The prevalence of adversarial examples raises questions about the reliability of machine learning systems, especially for their deployment in critical applications. Numerous defense mechanisms have been proposed that aim to improve a machine learning system’s robustness in the presence of adversarial examples. However, none of these methods are able to produce satisfactorily robust models, even for simple classification tasks on benchmarks. In addition to empirical attempts to build robust models, recent studies have identified intrinsic limitations for robust learning against adversarial examples. My research aims to gain a deeper understanding of why machine learning models fail in the presence of adversaries and design ways to build better robust systems. In this dissertation, I develop a concentration estimation framework to characterize the intrinsic limits of robustness for typical classification tasks of interest. The proposed framework leads to the discovery that compared with the concentration of measure which was previously argued to be an important factor, the existence of uncertain inputs may explain more fundamentally the vulnerability of state-of-the-art defenses. Moreover, to further advance our understanding of adversarial examples, I introduce a notion of representation robustness based on mutual information, which is shown to be related to an intrinsic limit of model robustness for downstream classification tasks. Finally in this dissertation, I advocate for a need to rethink the current design goal of robustness and shed light on ways to build better robust machine learning systems, potentially escaping the intrinsic limits of robustness.
Congratulations Dr. Xu!
Congratulations to Weilin Xu for successfully defending his PhD Thesis!
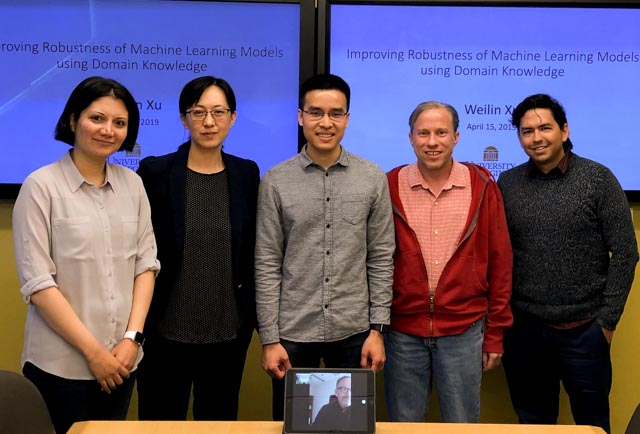
Although machine learning techniques have achieved great success in many areas, such as computer vision, natural language processing, and computer security, recent studies have shown that they are not robust under attack. A motivated adversary is often able to craft input samples that force a machine learning model to produce incorrect predictions, even if the target model achieves high accuracy on normal test inputs. This raises great concern when machine learning models are deployed for security-sensitive tasks.