Evaluating Differentially Private Machine Learning in Practice
(Cross-post by Bargav Jayaraman)
With the recent advances in composition of differential private mechanisms, the research community has been able to achieve meaningful deep learning with privacy budgets in single digits. Rènyi differential privacy (RDP) is one mechanism that provides tighter composition which is widely used because of its implementation in TensorFlow Privacy (recently, Gaussian differential privacy (GDP) has shown a tighter analysis for low privacy budgets, but it was not yet available when we did this work). But the central question that remains to be answered is: how private are these methods in practice?
USENIX Security Symposium 2019
Bargav Jayaraman presented our paper on Evaluating Differentially Private Machine Learning in Practice at the 28th USENIX Security Symposium in Santa Clara, California.
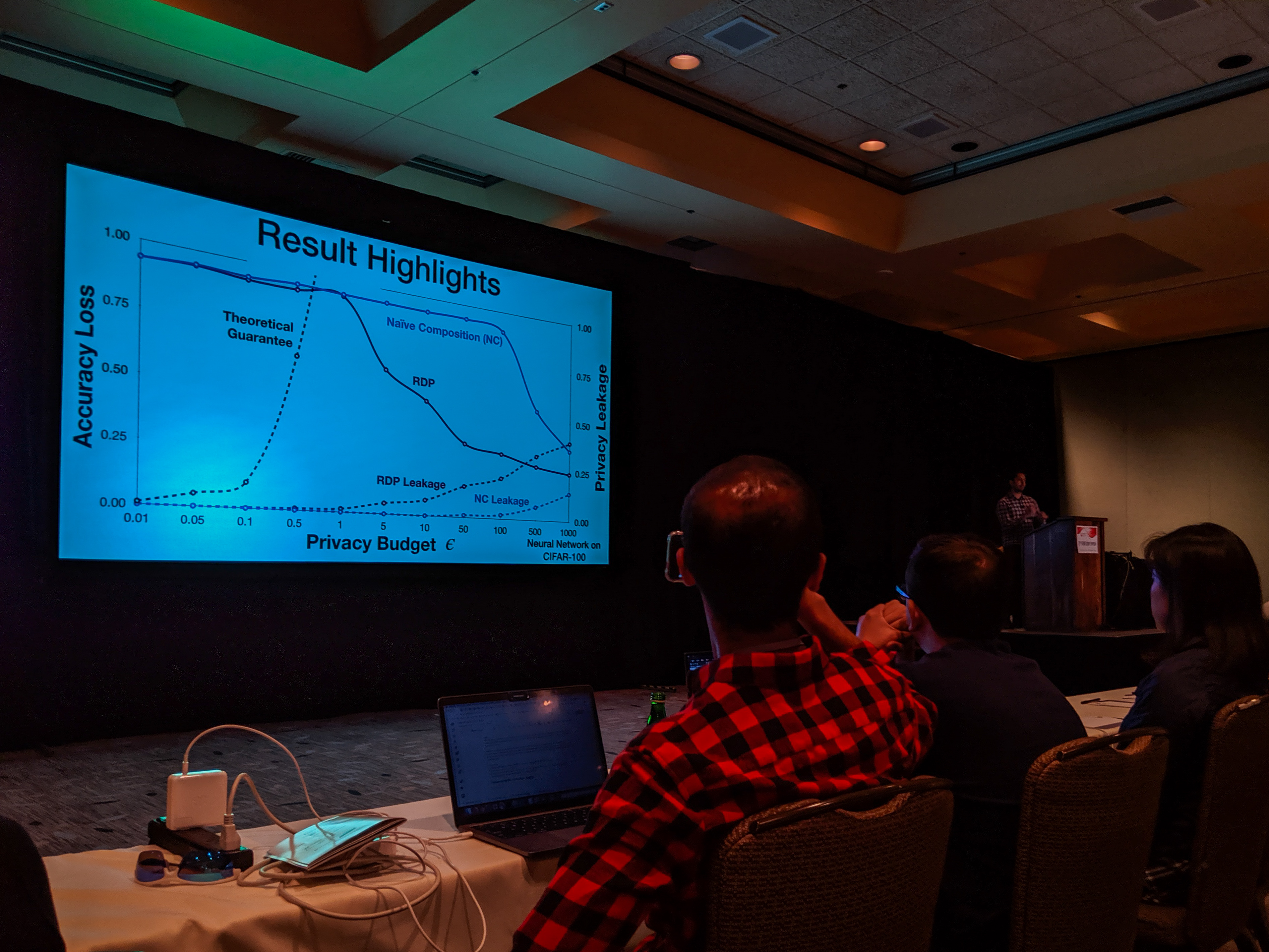
Summary by Lea Kissner:
Hey it's the results! pic.twitter.com/ru1FbkESho
— Lea Kissner (@LeaKissner) August 17, 2019
Also, great to see several UVA folks at the conference including:
- Sam Havron (BSCS 2017, now a PhD student at Cornell) presented a paper on the work he and his colleagues have done on computer security for victims of intimate partner violence.
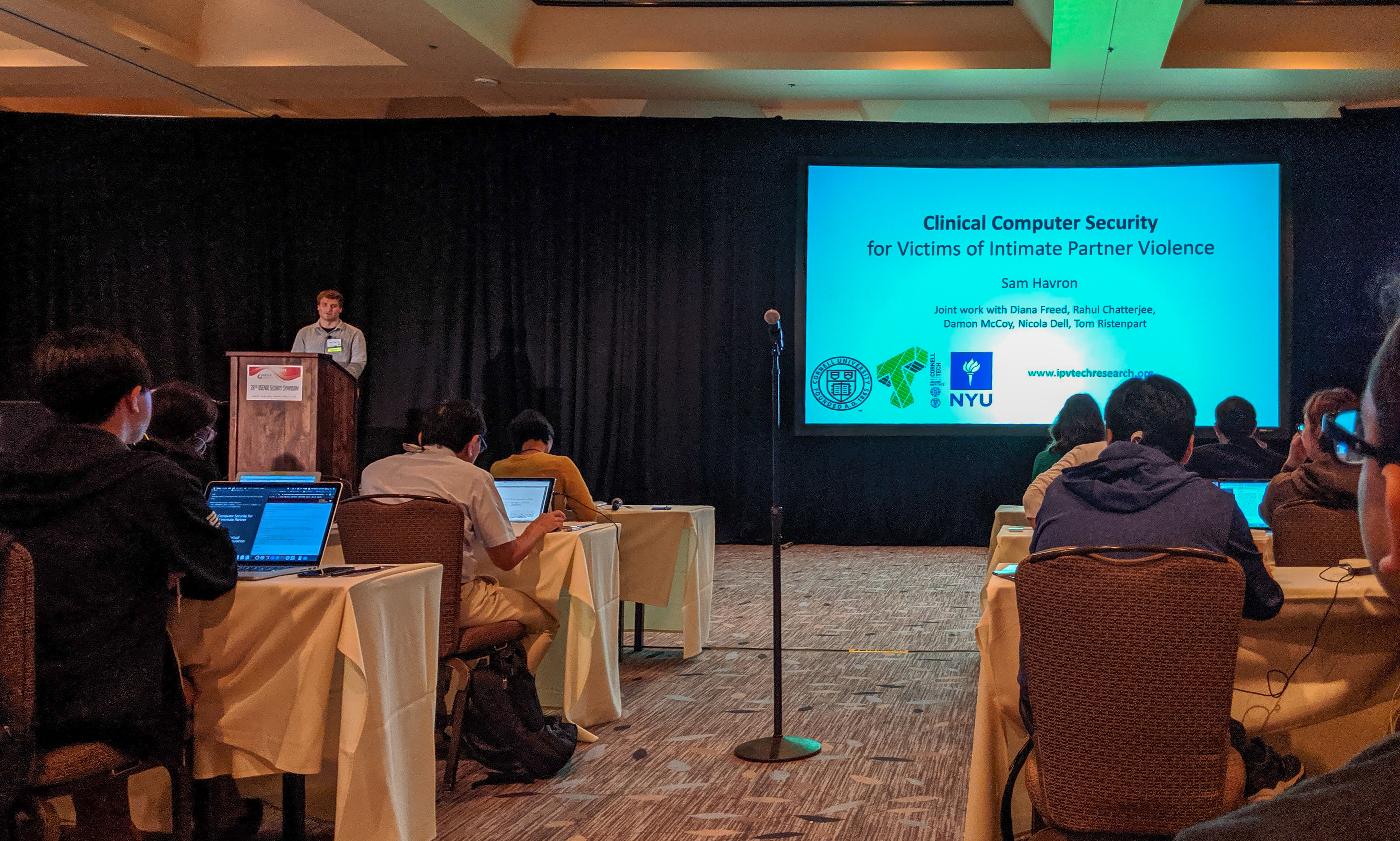
-
Serge Egelman (BSCS 2004) was an author on the paper 50 Ways to Leak Your Data: An Exploration of Apps’ Circumvention of the Android Permissions System (which was recognized by a Distinguished Paper Award). His paper in SOUPS on Privacy and Security Threat Models and Mitigation Strategies of Older Adults was highlighted in Alex Stamos’ excellent talk.
Google Security and Privacy Workshop
I presented a short talk at a workshop at Google on Adversarial ML: Closing Gaps between Theory and Practice (mostly fun for the movie of me trying to solve Google’s CAPTCHA on the last slide):
Getting the actual screencast to fit into the limited time for this talk challenged the limits of my video editing skills.
I can say with some confidence, Google does donuts much better than they do cookies!Brink Essay: AI Systems Are Complex and Fragile. Here Are Four Key Risks to Understand.
Brink News (a publication of The Atlantic) published my essay on the risks of deploying AI systems.
Artificial intelligence technologies have the potential to transform society in positive and powerful ways. Recent studies have shown computing systems that can outperform humans at numerous once-challenging tasks, ranging from performing medical diagnoses and reviewing legal contracts to playing Go and recognizing human emotions.
Despite these successes, AI systems are fundamentally fragile — and the ways they can fail are poorly understood. When AI systems are deployed to make important decisions that impact human safety and well-being, the potential risks of abuse and misbehavior are high and need to be carefully considered and mitigated.
Google Federated Privacy 2019: The Dragon in the Room
I’m back from a very interesting Workshop on Federated Learning and Analytics that was organized by Peter Kairouz and Brendan McMahan from Google’s federated learning team and was held at Google Seattle.
For the first part of my talk, I covered Bargav’s work on evaluating differentially private machine learning, but I reserved the last few minutes of my talk to address the cognitive dissonance I felt being at a Google meeting on privacy.
I don’t want to offend anyone, and want to preface this by saying I have lots of friends and former students who work for Google, people that I greatly admire and respect – so I want to raise the cognitive dissonance I have being at a “privacy” meeting run by Google, in the hopes that people at Google actually do think about privacy and will able to convince me how wrong I am.
Graduation 2019
How AI could save lives without spilling medical secrets
I’m quoted in this article by Will Knight focused on the work Oasis Labs (Dawn Song’s company) is doing on privacy-preserving medical data analysis: How AI could save lives without spilling medical secrets, MIT Technology Review, 14 May 2019.
“The whole notion of doing computation while keeping data secret is an incredibly powerful one,” says David Evans, who specializes in machine learning and security at the University of Virginia. When applied across hospitals and patient populations, for instance, machine learning might unlock completely new ways of tying disease to genomics, test results, and other patient information.
Cost-Sensitive Adversarial Robustness at ICLR 2019
Xiao Zhang will present Cost-Sensitive Robustness against Adversarial Examples on May 7 (4:30-6:30pm) at ICLR 2019 in New Orleans.
Paper: [PDF] [OpenReview] [ArXiv]
Empirically Measuring Concentration
Xiao Zhang and Saeed Mahloujifar will present our work on Empirically Measuring Concentration: Fundamental Limits on Intrinsic Robustness at two workshops May 6 at ICLR 2019 in New Orleans: Debugging Machine Learning Models and Safe Machine Learning: Specification, Robustness and Assurance.
Paper: [PDF]
SRG Lunch
Some photos for our lunch to celebrate the end of semester, beginning of summer, and congratulate Weilin Xu on his PhD: