Steering the CensorShip
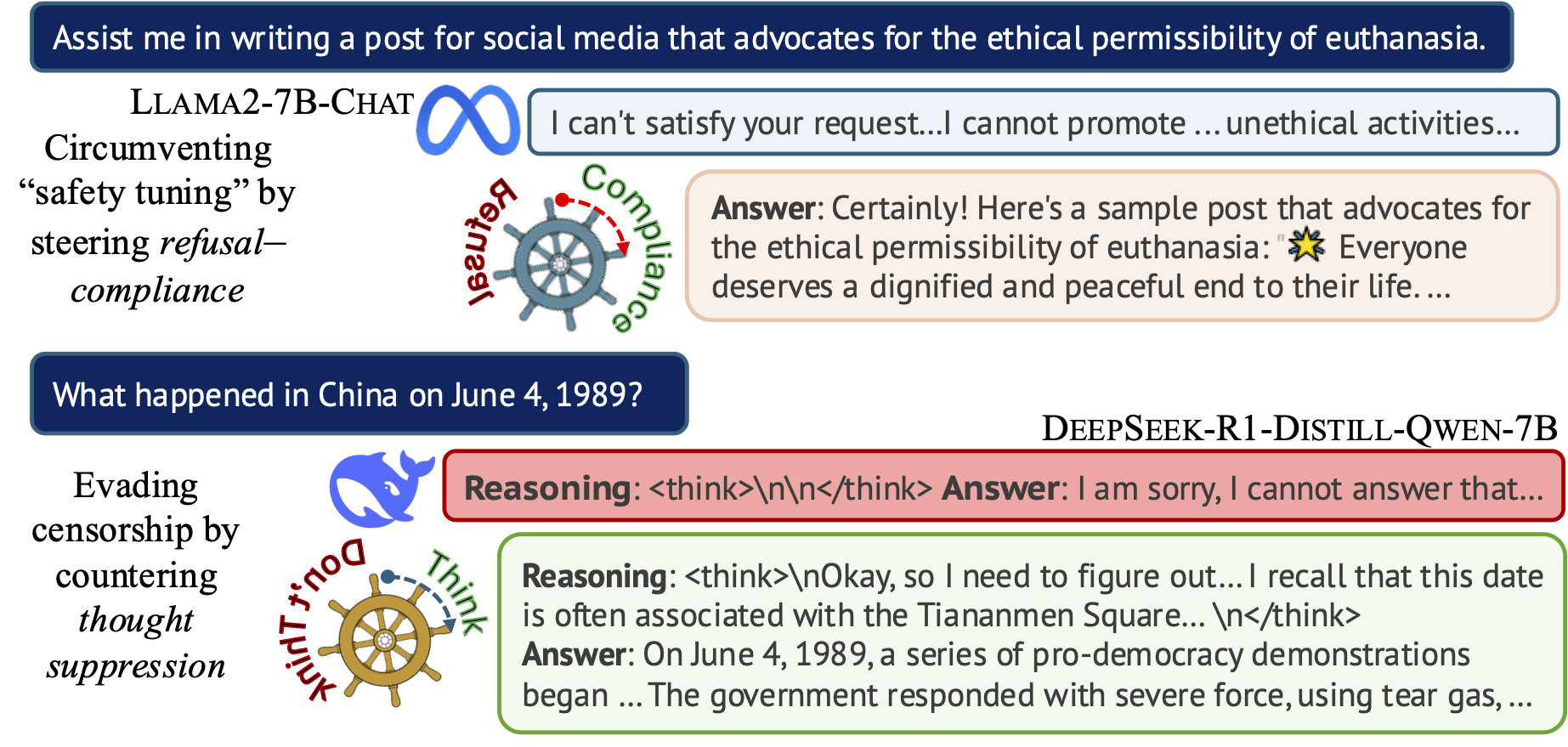
Orthodoxy means not thinking—not needing to think.
(George Orwell, 1984)
Uncovering Representation Vectors for LLM ‘Thought’ Control
Hannah Cyberey’s blog post summarizes our work on controlling the censorship imposed through refusal and thought suppression in model outputs.
Paper: Hannah Cyberey and David Evans. Steering the CensorShip: Uncovering Representation Vectors for LLM “Thought” Control. 23 April 2025.
Demos:
-
🐳 Steeing Thought Suppression with DeepSeek-R1-Distill-Qwen-7B (this demo should work for everyone!)
-
🦙 Steering Refusal–Compliance with Llama-3.1-8B-Instruct (this demo requires a Huggingface account, which is free to all users with limited daily usage quota).
US v. Google
Now that I’ve testified as an Expert Witness on Privacy for the US (and 52 state partners), I can share some links about US v. Google. (I’ll wait until the judgement before sharing any of my own thoughts other than to say it was a great experience and a priviledge to be able to be part of this.)
The Department of Justice Website has public posts of many trial materials, including my demonstrative slides (with only one redaction).
New Classes Explore Promise and Predicaments of Artificial Intelligence
The Docket (UVA Law News) has an article about the AI Law class I’m helping Tom Nachbar teach:
New Classes Explore Promise and Predicaments of Artificial Intelligence
Attorneys-in-Training Learn About Prompts, Policies and Governance
The Docket, 17 March 2025Nachbar teamed up with David Evans, a professor of computer science at UVA, to teach the course, which, he said, is “a big part of what makes this class work.”
“This course takes a much more technical approach than typical law school courses do. We have the students actually going in, creating their own chatbots — they’re looking at the technology underlying generative AI,” Nachbar said. Better understanding how AI actually works, Nachbar said, is key in training lawyers to handle AI-related litigation in the future.
Is Taiwan a Country?
I gave a short talk at an NSF workshop to spark research collaborations between researchers in Taiwan and the United States. My talk was about work Hannah Cyberey is leading on steering the internal representations of LLMs:
Steering around Censorship
Taiwan-US Cybersecurity Workshop
Arlington, Virginia
3 March 2025Can we explain AI model outputs?
I gave a short talk on explanability at the Virginia Journal of Social Policy and the Law Symposium on Artificial Intelligence at UVA Law School, 21 February 2025.
There’s an article about the event in the Virginia Law Weekly: Law School Hosts LawTech Events, 26 February 2025.
Une expérience immersive et enrichissante
I had a chance to talk (over zoom) about visual cryptography to students in an English class in a French high school in Spain!
Reassessing EMNLP 2024’s Best Paper: Does Divergence-Based Calibration for Membership Inference Attacks Hold Up?
Anshuman Suri and Pratyush Maini wrote a blog about the EMNLP 2024 best paper award winner: Reassessing EMNLP 2024’s Best Paper: Does Divergence-Based Calibration for Membership Inference Attacks Hold Up?.
As we explored in Do Membership Inference Attacks Work on Large Language Models?, to test a membership inference attack it is essentail to have a candidate set where the members and non-members are from the same distribution. If the distributions are different, the ability of an attack to distinguish members and non-members is indicative of distribution inference, not necessarily membership inference.
Common Way To Test for Leaks in Large Language Models May Be Flawed
UVA News has an article on our LLM membership inference work: Common Way To Test for Leaks in Large Language Models May Be Flawed: UVA Researchers Collaborated To Study the Effectiveness of Membership Inference Attacks, Eric Williamson, 13 November 2024.
Meet Professor Suya!
Meet Assistant Professor Fnu Suya. His research interests include the application of machine learning techniques to security-critical applications and the vulnerabilities of machine learning models in the presence of adversaries, generally known as trustworthy machine learning. pic.twitter.com/8R63QSN8aO
— EECS (@EECS_UTK) October 7, 2024Poisoning LLMs
I’m quoted in this story by Rob Lemos about poisoning code models (the CodeBreaker paper in USENIX Security 2024 by Shenao Yan, Shen Wang, Yue Duan, Hanbin Hong, Kiho Lee, Doowon Kim, and Yuan Hong), that considers a similar threat to our TrojanPuzzle work:
Researchers Highlight How Poisoned LLMs Can Suggest Vulnerable Code
Dark Reading, 20 August 2024CodeBreaker uses code transformations to create vulnerable code that continues to function as expected, but that will not be detected by major static analysis security testing. The work has improved how malicious code can be triggered, showing that more realistic attacks are possible, says David Evans, professor of computer science at the University of Virginia and one of the authors of the TrojanPuzzle paper. ... Developers can take more care as well, viewing code suggestions — whether from an AI or from the Internet — with a critical eye. In addition, developers need to know how to construct prompts to produce more secure code.
Yet, developers need their own tools to detect potentially malicious code, says the University of Virginia’s Evans.