Graduation 2024
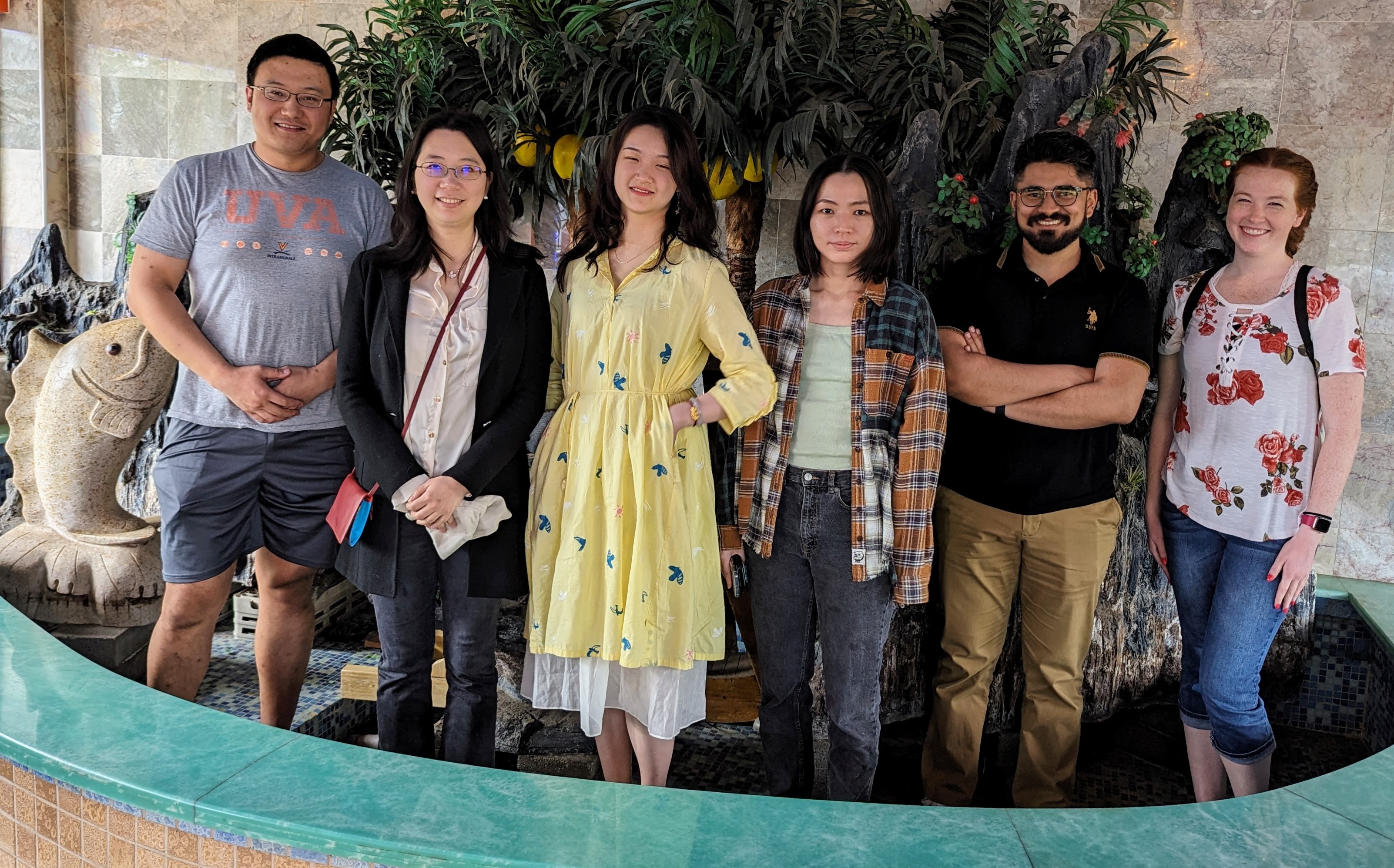
Congratulations to our two PhD graduates!
Suya will be joining the University of Tennessee at Knoxville as an Assistant Professor.
Josie will be building a medical analytics research group at Dexcom.
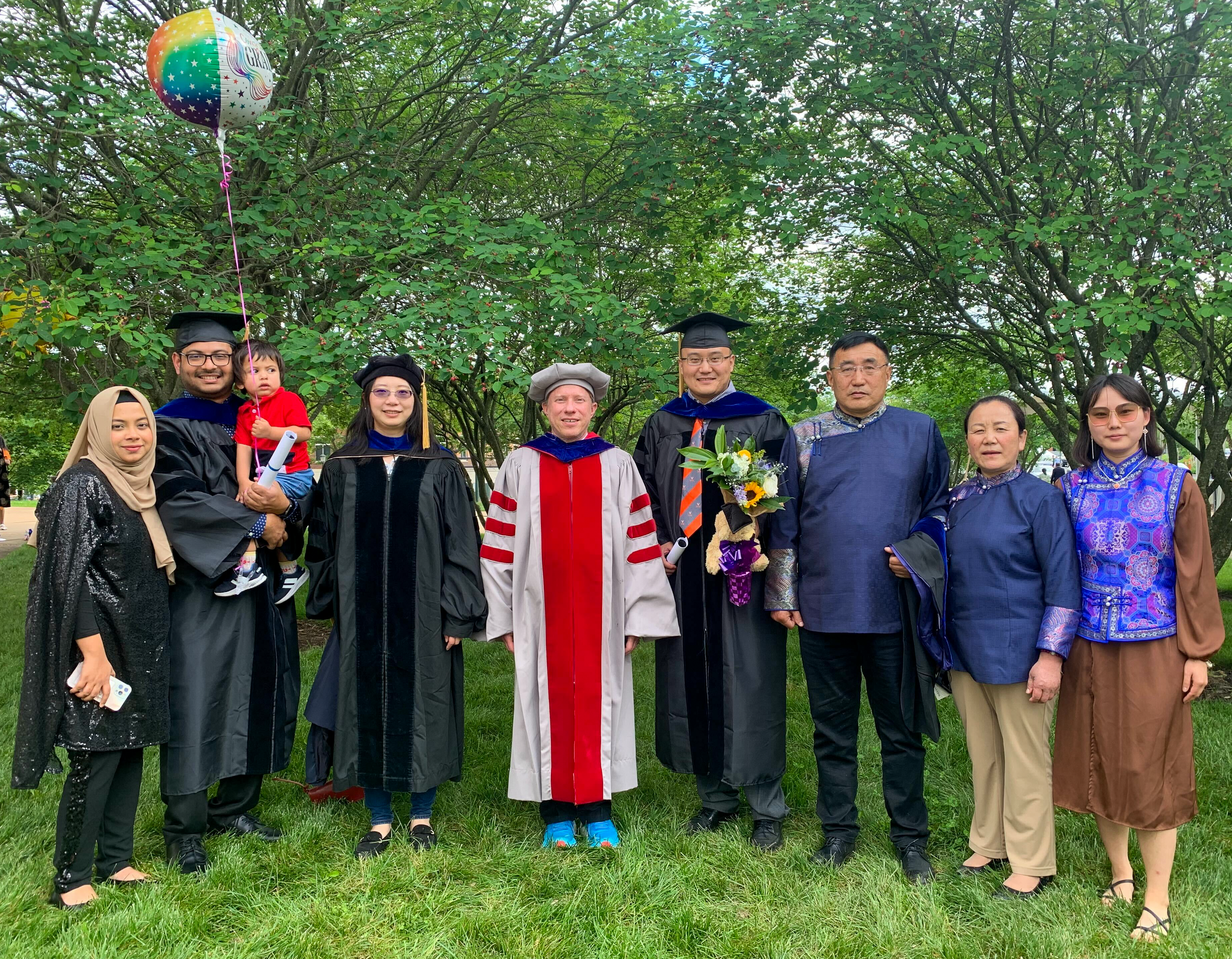
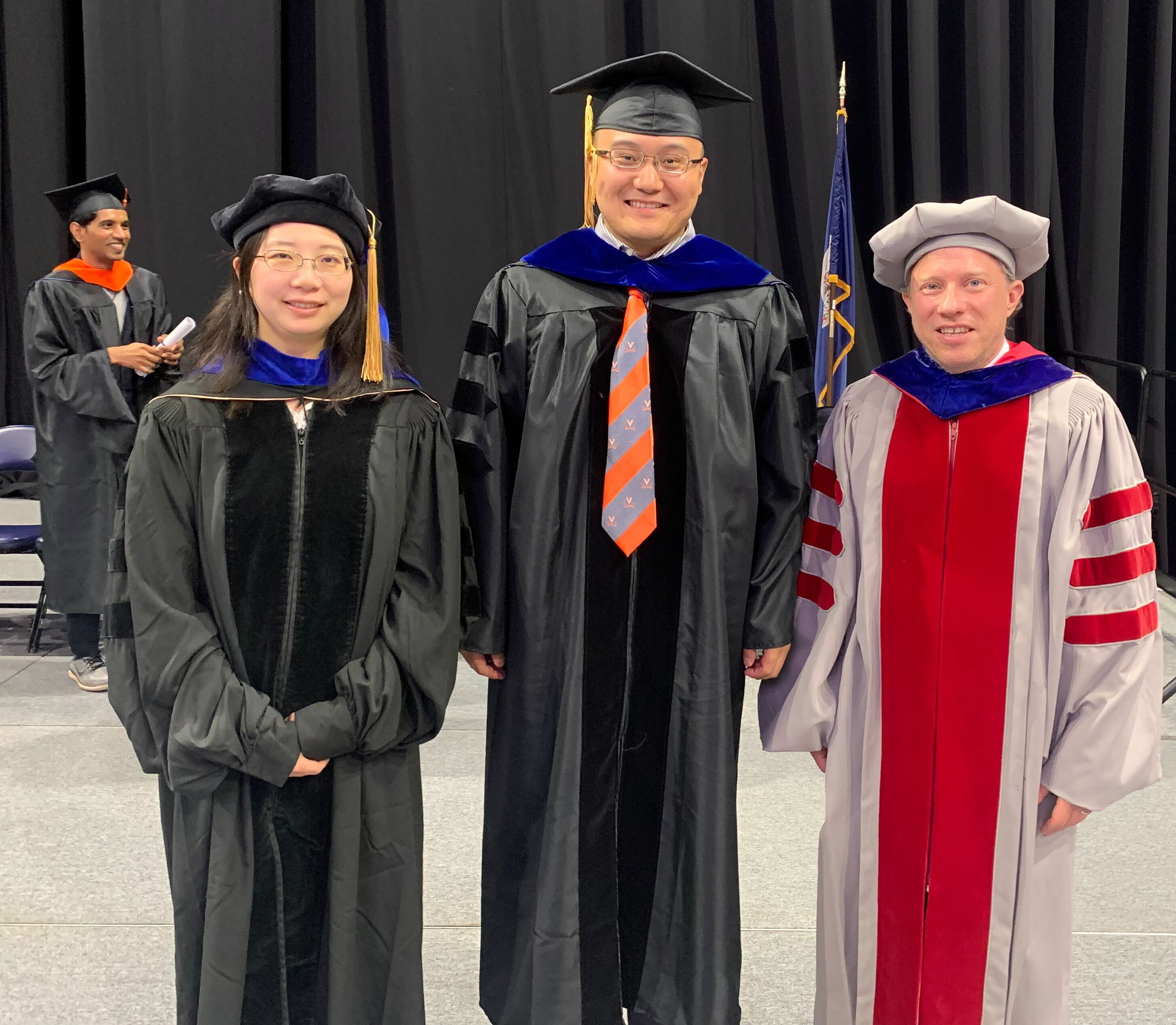
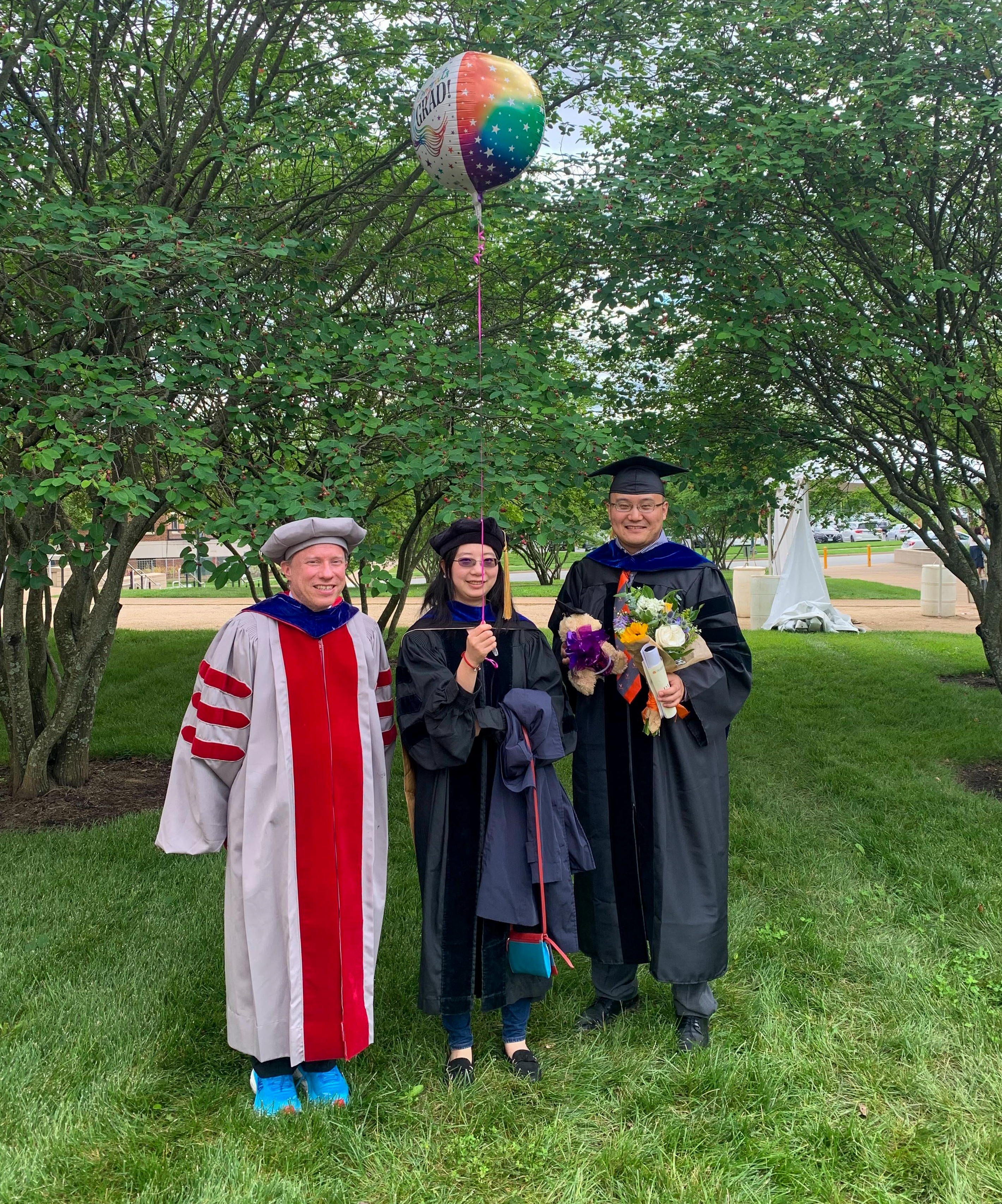
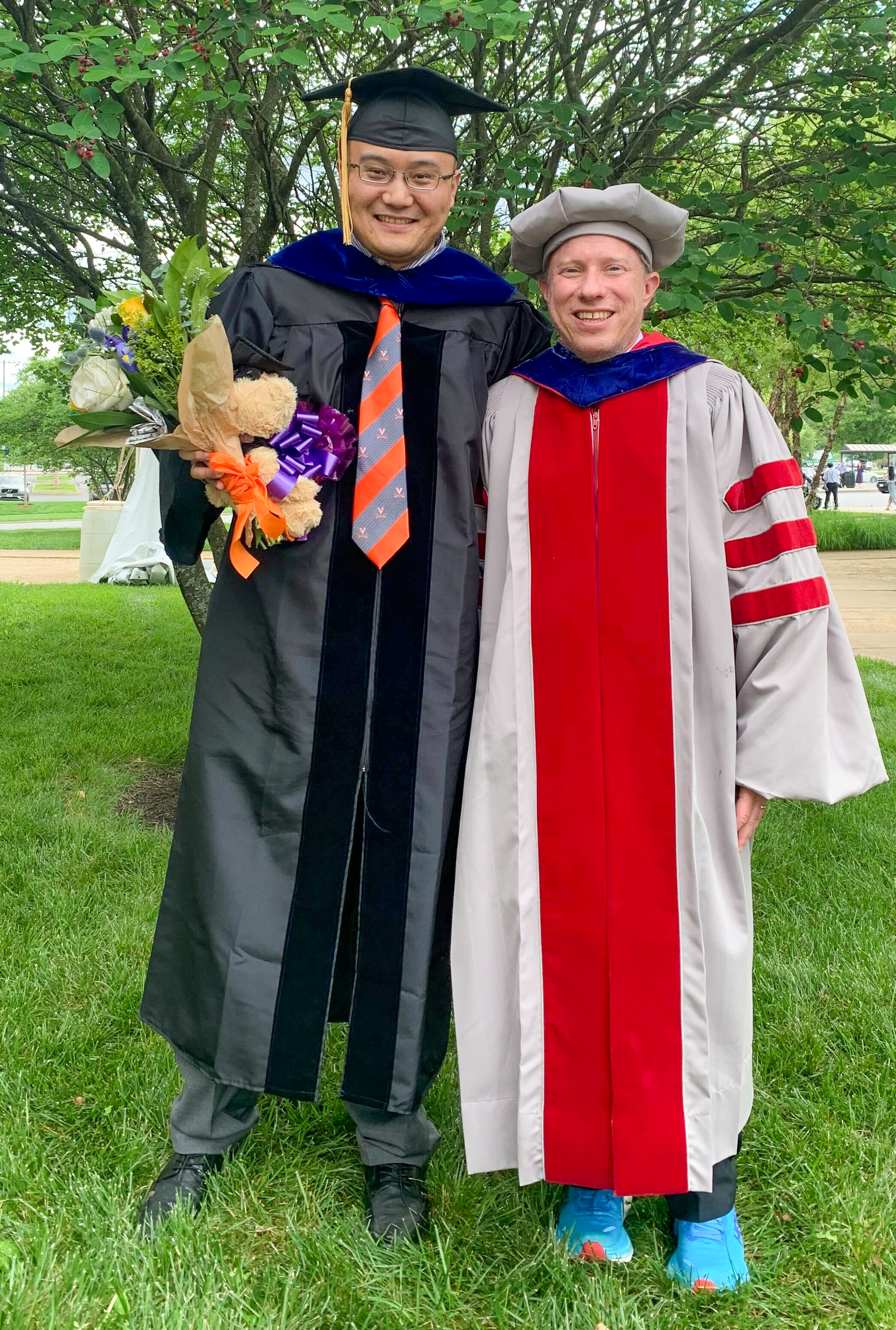
SaTML Talk: SoK: Pitfalls in Evaluating Black-Box Attacks
Anshuman Suri’s talk at IEEE Conference on Secure and Trustworthy Machine Learning (SaTML) is now available:
See the earlier blog post for more on the work, and the paper at https://arxiv.org/abs/2310.17534.
Congratulations, Dr. Lamp!
Tianhao Wang (Committee Chair), Miaomiao Zhang, Lu Feng (Co-Advisor), Dr. Josie Lamp, David Evans On screen: Sula Mazimba, Rich Nguyen, Tingting Zhu Congratulations to Josephine Lamp for successfully defending her PhD thesis! Trustworthy Clinical Decision Support Systems for Medical Trajectories The explosion of medical sensors and wearable devices has resulted in the collection of large amounts of medical trajectories. Medical trajectories are time series that provide a nuanced look into patient conditions and their changes over time, allowing for a more fine-grained understanding of patient health.Do Membership Inference Attacks Work on Large Language Models?
MIMIR logo. Image credit: GPT-4 + DALL-E Paper Code Data Membership inference attacks (MIAs) attempt to predict whether a particular datapoint is a member of a target model’s training data. Despite extensive research on traditional machine learning models, there has been limited work studying MIA on the pre-training data of large language models (LLMs). We perform a large-scale evaluation of MIAs over a suite of language models (LMs) trained on the Pile, ranging from 160M to 12B parameters.SoK: Pitfalls in Evaluating Black-Box Attacks
Post by Anshuman Suri and Fnu Suya Much research has studied black-box attacks on image classifiers, where adversaries generate adversarial examples against unknown target models without having access to their internal information. Our analysis of over 164 attacks (published in 102 major security, machine learning and security conferences) shows how these works make different assumptions about the adversary’s knowledge. The current literature lacks cohesive organization centered around the threat model. Our SoK paper (to appear at IEEE SaTML 2024) introduces a taxonomy for systematizing these attacks and demonstrates the importance of careful evaluations that consider adversary resources and threat models.NeurIPS 2023: What Distributions are Robust to Poisoning Attacks?
Post by Fnu Suya Data poisoning attacks are recognized as a top concern in the industry [1]. We focus on conventional indiscriminate data poisoning attacks, where an adversary injects a few crafted examples into the training data with the goal of increasing the test error of the induced model. Despite recent advances, indiscriminate poisoning attacks on large neural networks remain challenging [2]. In this work (to be presented at NeurIPS 2023), we revisit the vulnerabilities of more extensively studied linear models under indiscriminate poisoning attacks.Adjectives Can Reveal Gender Biases Within NLP Models
Post by Jason Briegel and Hannah Chen Because NLP models are trained with human corpora (and now, increasingly on text generated by other NLP models that were originally trained on human language), they are prone to inheriting common human stereotypes and biases. This is problematic, because with their growing prominence they may further propagate these stereotypes (Sun et al., 2019). For example, interest is growing in mitigating bias in the field of machine translation, where systems such as Google translate were observed to default to translating gender-neutral pronouns as male pronouns, even with feminine cues (Savoldi et al.Congratulations, Dr. Suya!
Congratulations to Fnu Suya for successfully defending his PhD thesis! Suya will join the Unversity of Maryland as a MC2 Postdoctoral Fellow at the Maryland Cybersecurity Center this fall. On the Limits of Data Poisoning Attacks Current machine learning models require large amounts of labeled training data, which are often collected from untrusted sources. Models trained on these potentially manipulated data points are prone to data poisoning attacks. My research aims to gain a deeper understanding on the limits of two types of data poisoning attacks: indiscriminate poisoning attacks, where the attacker aims to increase the test error on the entire dataset; and subpopulation poisoning attacks, where the attacker aims to increase the test error on a defined subset of the distribution.SoK: Let the Privacy Games Begin! A Unified Treatment of Data Inference Privacy in Machine Learning
Our paper on the use of cryptographic-style games to model inference privacy is published in IEEE Symposium on Security and Privacy (Oakland):
Giovanni Cherubin, , Boris Köpf, Andrew Paverd, Anshuman Suri, Shruti Tople, and Santiago Zanella-Béguelin. SoK: Let the Privacy Games Begin! A Unified Treatment of Data Inference Privacy in Machine Learning. IEEE Symposium on Security and Privacy, 2023. [Arxiv]
Tired of diverse definitions of machine learning privacy risks? Curious about game-based definitions? In our paper, we present privacy games as a tool for describing and analyzing privacy risks in machine learning. Join us on May 22nd, 11 AM @IEEESSP '23 https://t.co/NbRuTmHyd2 pic.twitter.com/CIzsT7UY4b
— ahmed salem (@AhmedGaSalem) May 15, 2023