Research Symposium Posters
Five students from our group presented posters at the department’s Fall Research Symposium:
Anshuman Suri's Overview Talk
Cantor's (No Longer) Lost Proof
In preparing to cover Cantor’s proof of different infinite set cardinalities (one of my all-time favorite topics!) in our theory of computation course, I found various conflicting accounts of what Cantor originally proved. So, I figured it would be easy to search the web to find the original proof. Shockingly, at least as far as I could find1, it didn’t exist on the web! The closest I could find was in Google Books the 1892 volume of the Jähresbericht Deutsche Mathematiker-Vereinigung (which many of the references pointed to), but in fact not the first value of that journal which contains the actual proof.FOSAD Trustworthy Machine Learning Mini-Course
I taught a mini-course on Trustworthy Machine Learning at the 19th International School on Foundations of Security Analysis and Design in Bertinoro, Italy. Slides from my three (two-hour) lectures are posted below, along with some links to relevant papers and resources. Class 1: Introduction/Attacks The PDF malware evasion attack is described in this paper: Weilin Xu, Yanjun Qi, and David Evans. Automatically Evading Classifiers: A Case Study on PDF Malware Classifiers.Evaluating Differentially Private Machine Learning in Practice
(Cross-post by Bargav Jayaraman) With the recent advances in composition of differential private mechanisms, the research community has been able to achieve meaningful deep learning with privacy budgets in single digits. Rènyi differential privacy (RDP) is one mechanism that provides tighter composition which is widely used because of its implementation in TensorFlow Privacy (recently, Gaussian differential privacy (GDP) has shown a tighter analysis for low privacy budgets, but it was not yet available when we did this work).USENIX Security Symposium 2019
Bargav Jayaraman presented our paper on Evaluating Differentially Private Machine Learning in Practice at the 28th USENIX Security Symposium in Santa Clara, California. Summary by Lea Kissner: Hey it's the results! pic.twitter.com/ru1FbkESho — Lea Kissner (@LeaKissner) August 17, 2019 Also, great to see several UVA folks at the conference including: Sam Havron (BSCS 2017, now a PhD student at Cornell) presented a paper on the work he and his colleagues have done on computer security for victims of intimate partner violence.Google Security and Privacy Workshop
I presented a short talk at a workshop at Google on Adversarial ML: Closing Gaps between Theory and Practice (mostly fun for the movie of me trying to solve Google’s CAPTCHA on the last slide): Getting the actual screencast to fit into the limited time for this talk challenged the limits of my video editing skills. I can say with some confidence, Google does donuts much better than they do cookies!Brink Essay: AI Systems Are Complex and Fragile. Here Are Four Key Risks to Understand.
Brink News (a publication of The Atlantic) published my essay on the risks of deploying AI systems. Artificial intelligence technologies have the potential to transform society in positive and powerful ways. Recent studies have shown computing systems that can outperform humans at numerous once-challenging tasks, ranging from performing medical diagnoses and reviewing legal contracts to playing Go and recognizing human emotions. Despite these successes, AI systems are fundamentally fragile — and the ways they can fail are poorly understood.Google Federated Privacy 2019: The Dragon in the Room
I’m back from a very interesting Workshop on Federated Learning and Analytics that was organized by Peter Kairouz and Brendan McMahan from Google’s federated learning team and was held at Google Seattle. For the first part of my talk, I covered Bargav’s work on evaluating differentially private machine learning, but I reserved the last few minutes of my talk to address the cognitive dissonance I felt being at a Google meeting on privacy.Graduation 2019
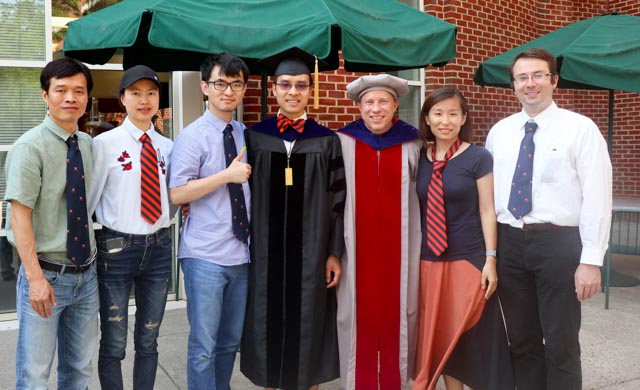
![]() |
![]() |
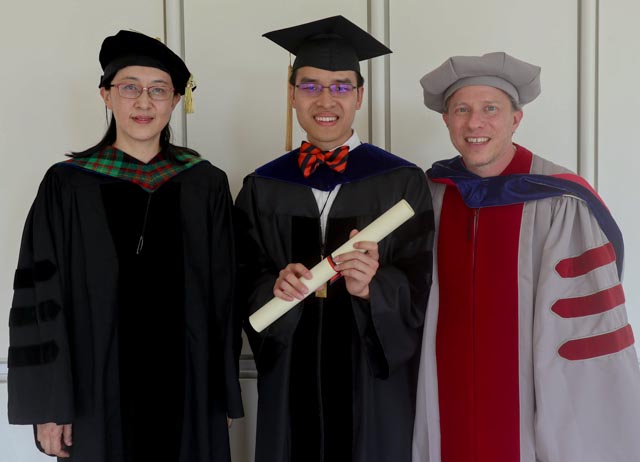