Codaspy 2021 Keynote: When Models Learn Too Much
Here are the slides for my talk at the 11th ACM Conference on Data and Application Security and Privacy: When Models Learn Too Much [PDF] The talk includes Bargav Jayaraman’s work (with Katherine Knipmeyer, Lingxiao Wang, and Quanquan Gu) on evaluating privacy in machine learning, as well as more recent work by Anshuman Suri on property inference attacks, and Bargav on attribute inference and imputation: Merlin, Morgan, and the Importance of Thresholds and Priors Evaluating Differentially Private Machine Learning in Practice “When models learn too much.CrySP Talk: When Models Learn Too Much
I gave a talk on When Models Learn Too Much at the University of Waterloo (virtually) in the CrySP Speaker Series on Privacy (29 March 2021): Abstract Statistical machine learning uses training data to produce models that capture patterns in that data. When models are trained on private data, such as medical records or personal emails, there is a risk that those models not only learn the hoped-for patterns, but will also learn and expose sensitive information about their training data.Improved Estimation of Concentration (ICLR 2021)
Our paper on Improved Estimation of Concentration Under ℓp-Norm Distance Metrics Using Half Spaces (Jack Prescott, Xiao Zhang, and David Evans) will be presented at ICLR 2021. Abstract: Concentration of measure has been argued to be the fundamental cause of adversarial vulnerability. Mahloujifar et al. (2019) presented an empirical way to measure the concentration of a data distribution using samples, and employed it to find lower bounds on intrinsic robustness for several benchmark datasets.Virginia Consumer Data Protection Act
Josephine Lamp presented on the new data privacy law that is pending in Virginia (it still needs a few steps including expected signing by governor, but likely to go into effect Jan 1, 2023): Slides (PDF)
This article provides a summary of the law: Virginia Passes Consumer Privacy Law; Other States May Follow, National Law Review, 17 February 2021.
The law itself is here: SB 1392: Consumer Data Protection Act
Algorithmic Accountability and the Law
Brink News (a publication of The Atlantic) published an essay I co-authored with Tom Nachbar (UVA Law School) on how the law views algorithmic accountability and the limits of what measures are permitted under the law to adjust algorithms to counter inequity: Algorithms Are Running Foul of Anti-Discrimination Law Tom Nachbar and David Evans Brink, 7 December 2020 Computing systems that are found to discriminate on prohibited bases, such as race or sex, are no longer surprising.Microsoft Security Data Science Colloquium: Inference Privacy in Theory and Practice
Here are the slides for my talk at the Microsoft Security Data Science Colloquium:
When Models Learn Too Much: Inference Privacy in Theory and Practice [PDF]
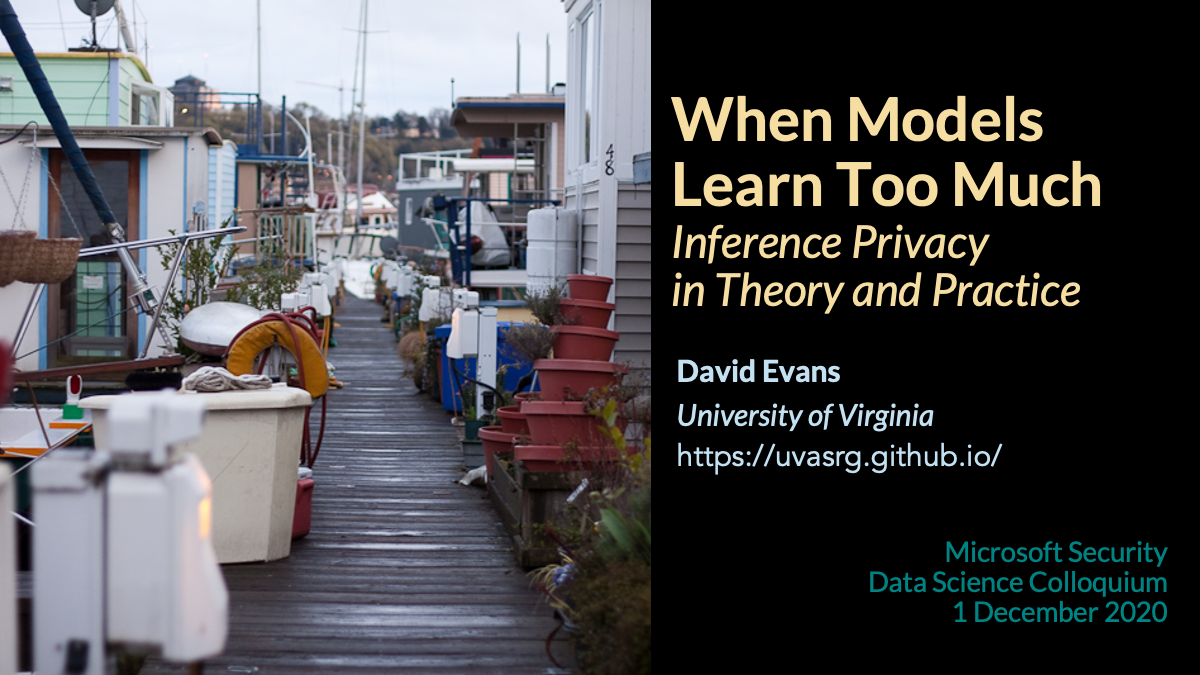
The talk is mostly about Bargav Jayaraman’s work (with Katherine Knipmeyer, Lingxiao Wang, and Quanquan Gu) on evaluating privacy:
- Merlin, Morgan, and the Importance of Thresholds and Priors
- Evaluating Differentially Private Machine Learning in Practice
Fact-checking Donald Trump’s tweet firing Christopher Krebs
I was a source for thie “Pants on Fire!” fact check by PolitiFact on Donald Trump’s tweet that fired Christopher Krebs claiming that “The recent statement by Chris Krebs on the security of the 2020 Election was highly inaccurate, in that there were massive improprieties and fraud - including dead people voting, Poll Watchers not allowed into polling locations, “glitches” in the voting machines which changed…” PolitiFact: Fact-checking Donald Trump’s tweet firing Christopher Krebs, 18 November 2020Voting Security
I was interviewed for a local news story by Daniel Grimes on election security: UVA cybersecurity expert: Virginia is one of the safer states to cast a ballot, NBC 29 News, 21 October 2020.