Graduation 2024
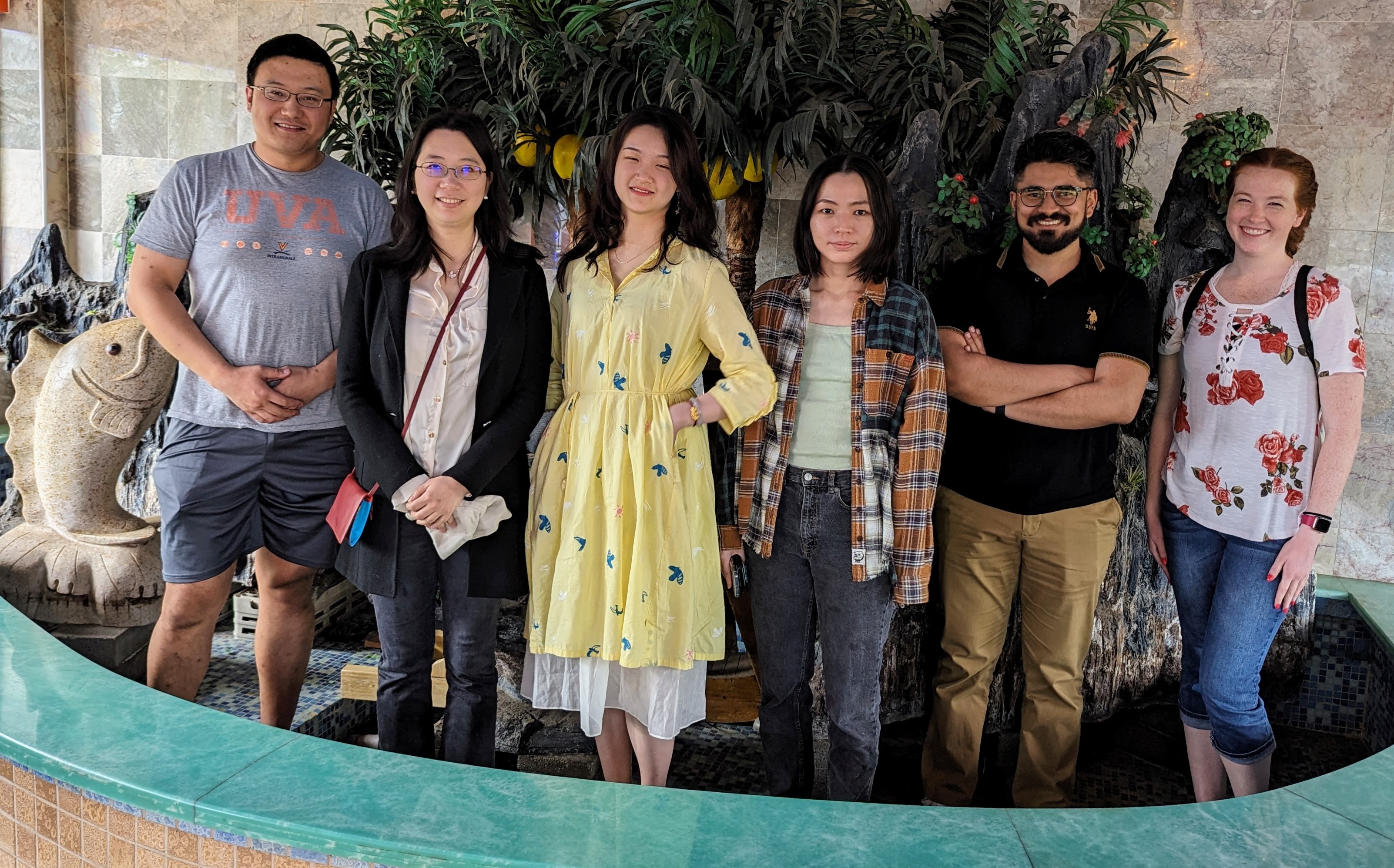
Congratulations to our two PhD graduates!
Suya will be joining the University of Tennessee at Knoxville as an Assistant Professor.
Josie will be building a medical analytics research group at Dexcom.
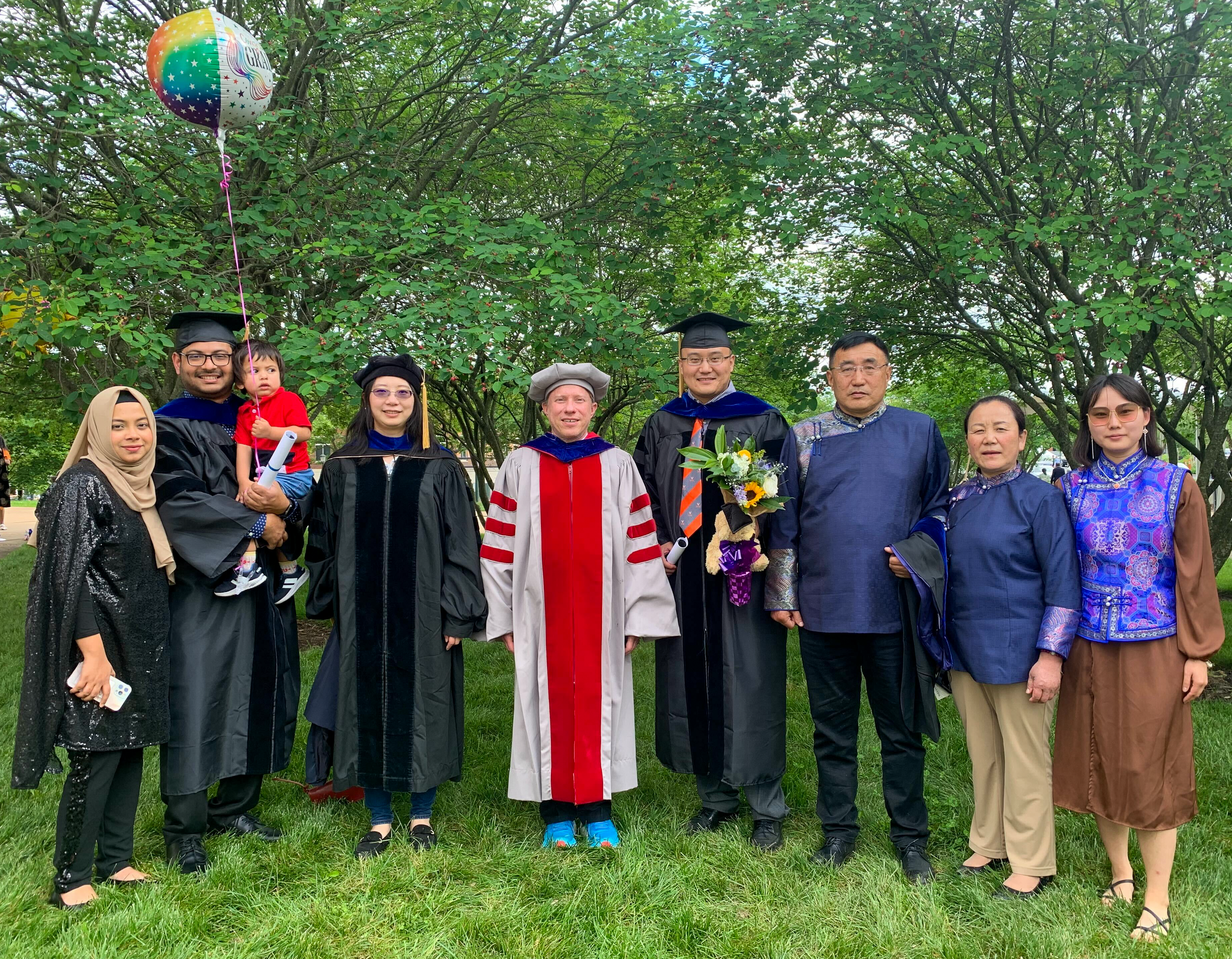
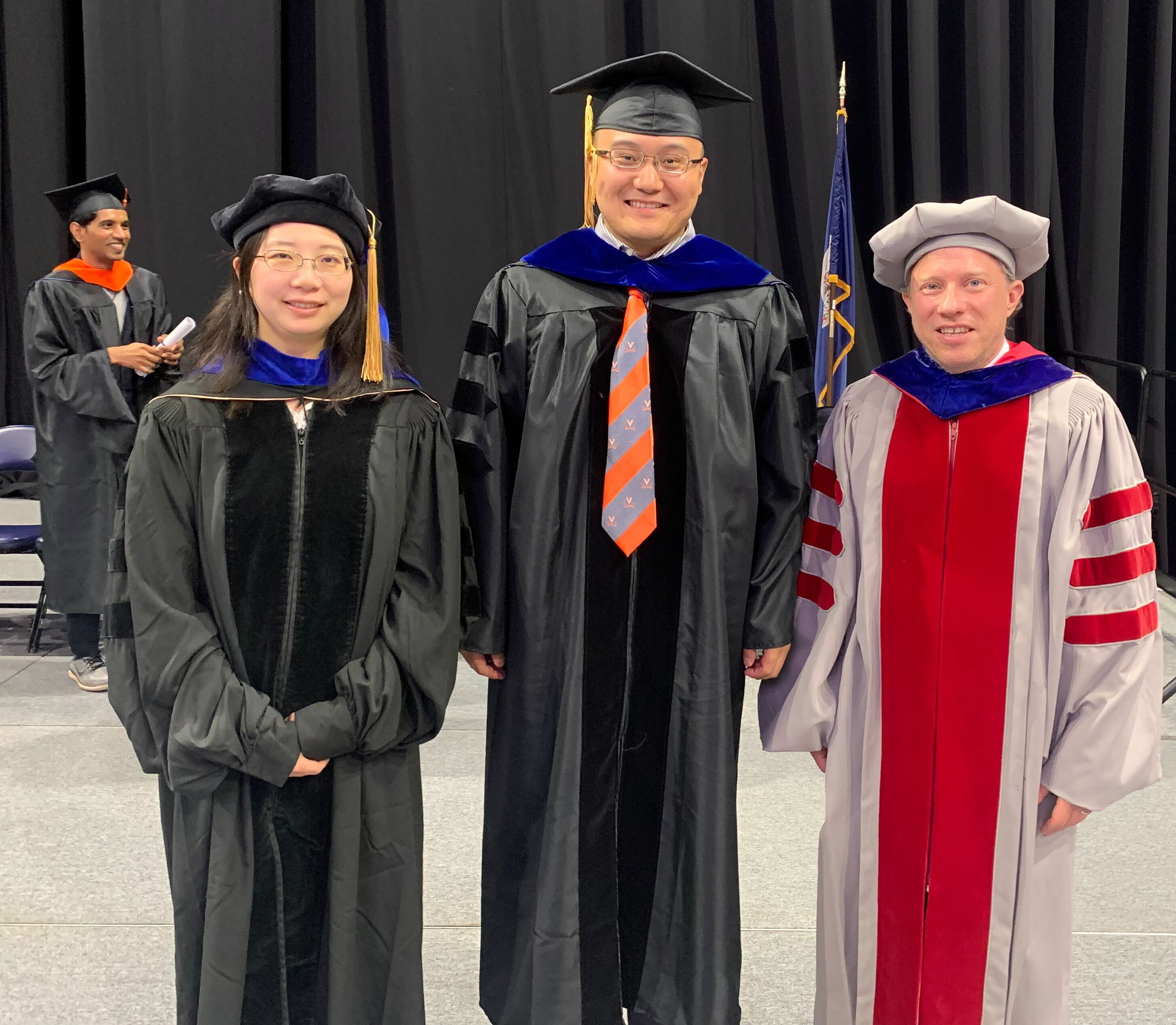
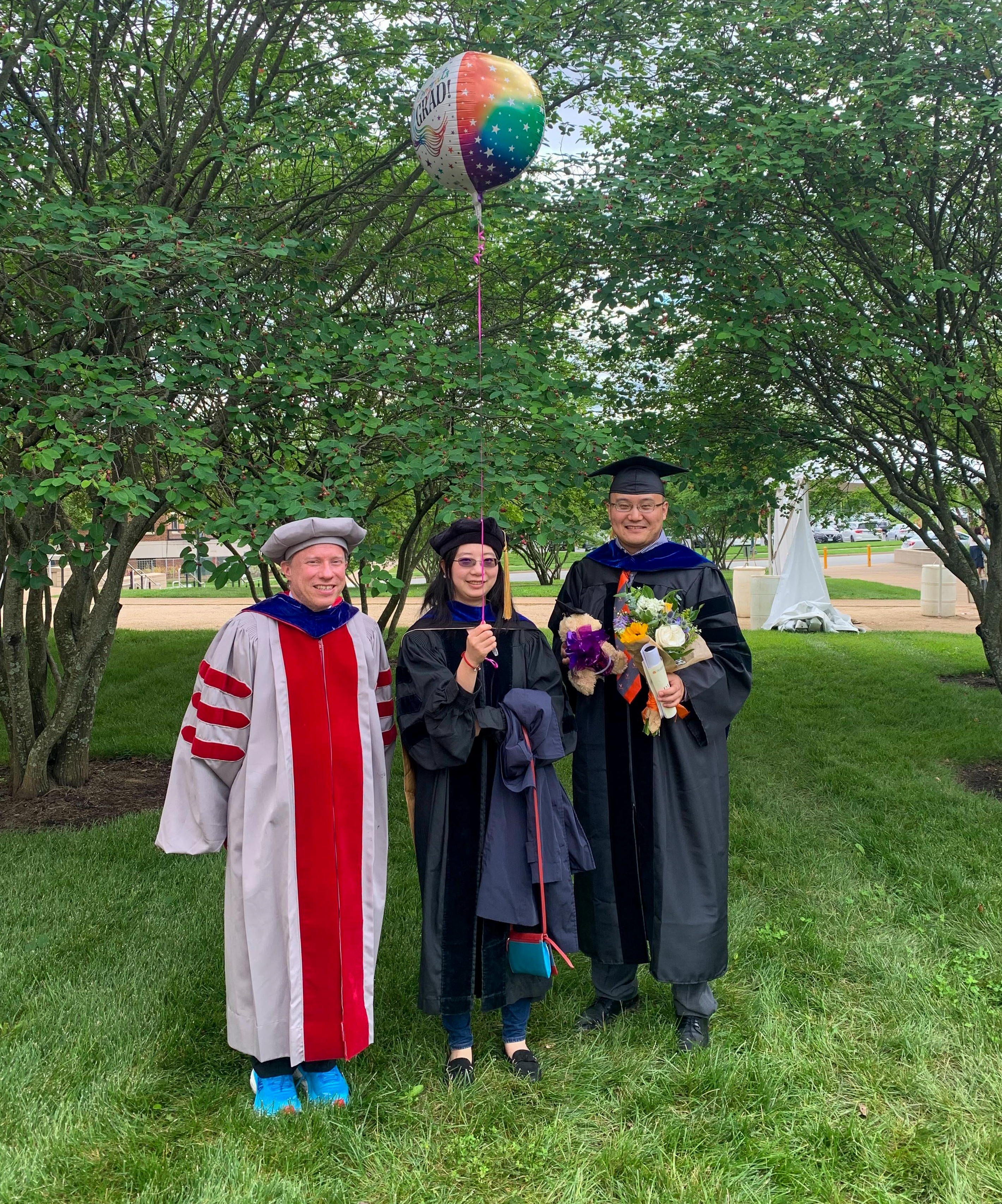
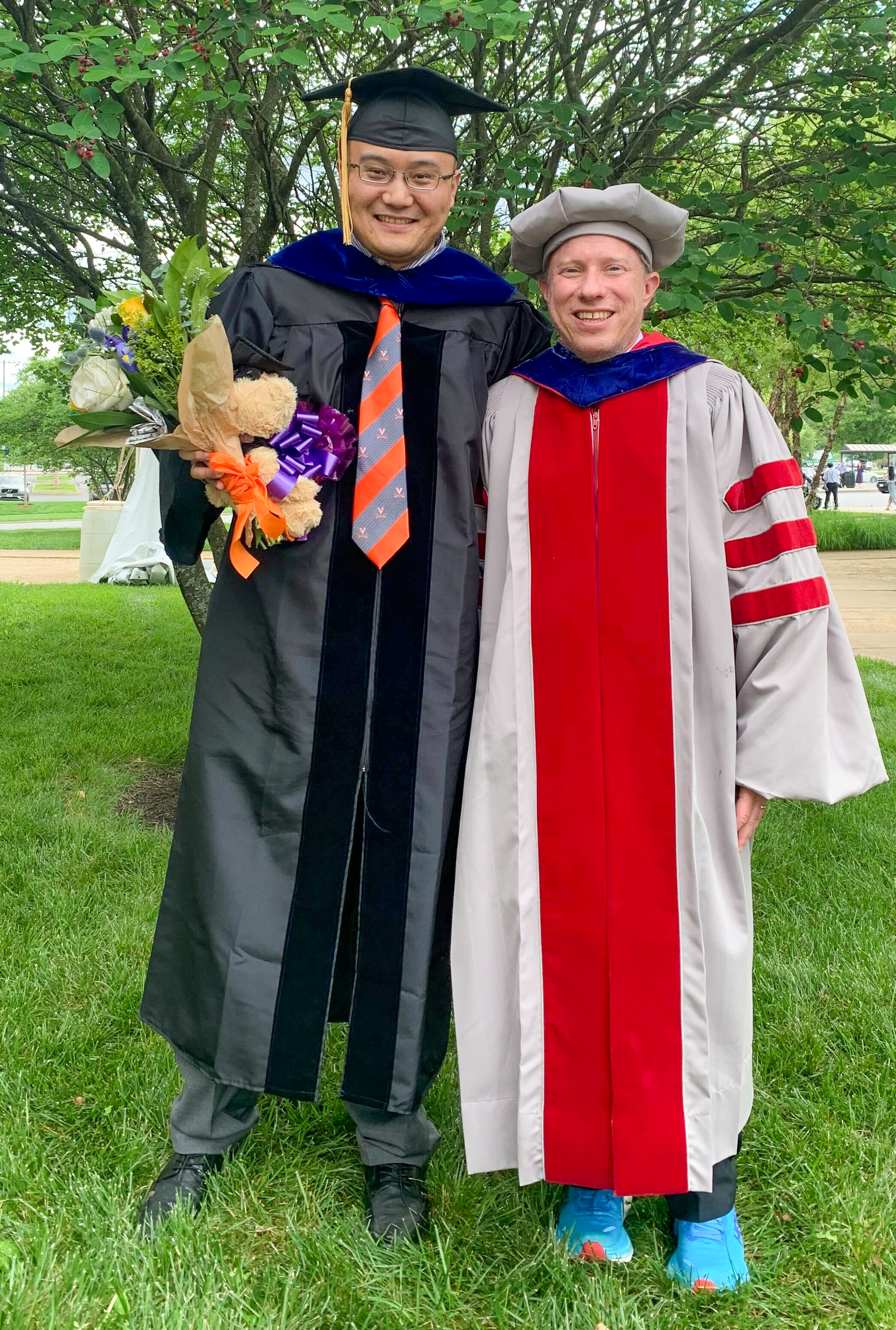
SaTML Talk: SoK: Pitfalls in Evaluating Black-Box Attacks
Anshuman Suri’s talk at IEEE Conference on Secure and Trustworthy Machine Learning (SaTML) is now available:
See the earlier blog post for more on the work, and the paper at https://arxiv.org/abs/2310.17534.
SoK: Pitfalls in Evaluating Black-Box Attacks
Post by Anshuman Suri and Fnu Suya Much research has studied black-box attacks on image classifiers, where adversaries generate adversarial examples against unknown target models without having access to their internal information. Our analysis of over 164 attacks (published in 102 major security, machine learning and security conferences) shows how these works make different assumptions about the adversary’s knowledge. The current literature lacks cohesive organization centered around the threat model. Our SoK paper (to appear at IEEE SaTML 2024) introduces a taxonomy for systematizing these attacks and demonstrates the importance of careful evaluations that consider adversary resources and threat models.NeurIPS 2023: What Distributions are Robust to Poisoning Attacks?
Post by Fnu Suya Data poisoning attacks are recognized as a top concern in the industry [1]. We focus on conventional indiscriminate data poisoning attacks, where an adversary injects a few crafted examples into the training data with the goal of increasing the test error of the induced model. Despite recent advances, indiscriminate poisoning attacks on large neural networks remain challenging [2]. In this work (to be presented at NeurIPS 2023), we revisit the vulnerabilities of more extensively studied linear models under indiscriminate poisoning attacks.Congratulations, Dr. Suya!
Congratulations to Fnu Suya for successfully defending his PhD thesis! Suya will join the Unversity of Maryland as a MC2 Postdoctoral Fellow at the Maryland Cybersecurity Center this fall. On the Limits of Data Poisoning Attacks Current machine learning models require large amounts of labeled training data, which are often collected from untrusted sources. Models trained on these potentially manipulated data points are prone to data poisoning attacks. My research aims to gain a deeper understanding on the limits of two types of data poisoning attacks: indiscriminate poisoning attacks, where the attacker aims to increase the test error on the entire dataset; and subpopulation poisoning attacks, where the attacker aims to increase the test error on a defined subset of the distribution.CVPR 2023: Manipulating Transfer Learning for Property Inference
Manipulating Transfer Learning for Property Inference Transfer learning is a popular method to train deep learning models efficiently. By reusing parameters from upstream pre-trained models, the downstream trainer can use fewer computing resources to train downstream models, compared to training models from scratch. The figure below shows the typical process of transfer learning for vision tasks: However, the nature of transfer learning can be exploited by a malicious upstream trainer, leading to severe risks to the downstream trainer.Visualizing Poisoning
How does a poisoning attack work and why are some groups more susceptible to being victimized by a poisoning attack? We’ve posted work that helps understand how poisoning attacks work with some engaging visualizations: Poisoning Attacks and Subpopulation Susceptibility An Experimental Exploration on the Effectiveness of Poisoning Attacks Evan Rose, Fnu Suya, and David Evans Follow the link to try the interactive version! Machine learning is susceptible to poisoning attacks in which adversaries inject maliciously crafted training data into the training set to induce specific model behavior.Model-Targeted Poisoning Attacks with Provable Convergence
(Post by Sean Miller, using images adapted from Suya’s talk slides) Data Poisoning Attacks Machine learning models are often trained using data from untrusted sources, leaving them open to poisoning attacks where adversaries use their control over a small fraction of that training data to poison the model in a particular way. Most work on poisoning attacks is directly driven by an attacker’s objective, where the adversary chooses poisoning points that maximize some target objective.How to Hide a Backdoor
The Register has an article on our recent work on Stealthy Backdoors as Compression Artifacts: Thomas Claburn, How to hide a backdoor in AI software — Neural networks can be aimed to misbehave when squeezed, The Register, 5 May 2021.
Hybrid Batch Attacks at USENIX Security 2020
Here’s the video for Suya’s presentation on Hybrid Batch Attacks at USENIX Security 2020:
Download Video [mp4]