David Evans, professor of computer science in the University of Virginia School of Engineering and Applied Science, is leading research to understand how machine learning models can be compromised.
Model-Targeted Poisoning Attacks with Provable Convergence
(Post by Sean Miller, using images adapted from Suya’s talk slides)
Data Poisoning Attacks
Machine learning models are often trained using data from untrusted sources, leaving them open to poisoning attacks where adversaries use their control over a small fraction of that training data to poison the model in a particular way.
Most work on poisoning attacks is directly driven by an attacker’s objective, where the adversary chooses poisoning points that maximize some target objective. Our work focuses on model-targeted poisoning attacks, where the adversary splits the attack into choosing a target model that satisfies the objective and then choosing poisoning points that induce the target model.
On the Risks of Distribution Inference
(Cross-post by Anshuman Suri)
Inference attacks seek to infer sensitive information about the training process of a revealed machine-learned model, most often about the training data.
Standard inference attacks (which we call ādataset inference attacksā) aim to learn something about a particular record that may have been in that training data. For example, in a membership inference attack (Reza Shokri et al., Membership Inference Attacks Against Machine Learning Models, IEEE S&P 2017), the adversary aims to infer whether or not a particular record was included in the training data.
Chinese Translation of MPC Book
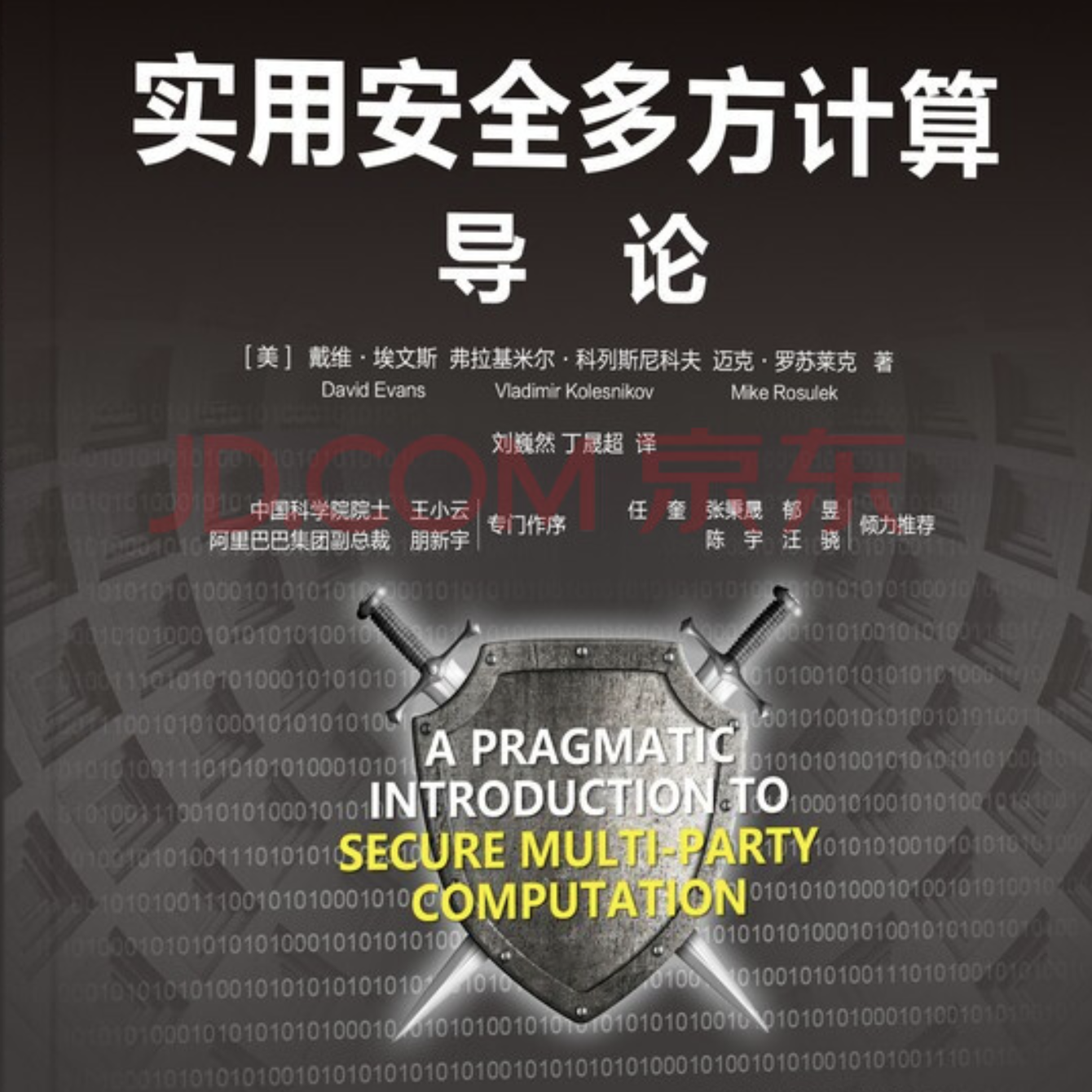
A Chinese translation of our A Pragmatic Introduction to Secure Multi-Party Computation book (by David Evans, Vladimir Kolesnikov, and Mike Rosulek) is now available!
Thanks to Weiran Liu and Sengchao Ding for all the work they did on the translation.
To order from JD.com: https://item.jd.com/13302742.html
(The English version of the book is still available for free download, from https://securecomputation.org.)